mirror of
https://github.com/Shawn-Shan/fawkes.git
synced 2024-09-19 01:16:37 +05:30
1.0 beta update
This commit is contained in:
parent
63ba2f9b73
commit
cfb34e1d39
50
README.md
50
README.md
@ -2,12 +2,13 @@ Fawkes
|
||||
------
|
||||
:warning: Check out our MacOS/Windows Software on our official [webpage](https://sandlab.cs.uchicago.edu/fawkes/#code).
|
||||
|
||||
Fawkes is a privacy protection system developed by researchers at [SANDLab](https://sandlab.cs.uchicago.edu/), University of Chicago. For more information about the project, please refer to our project [webpage](https://sandlab.cs.uchicago.edu/fawkes/). Contact us at fawkes-team@googlegroups.com.
|
||||
|
||||
We published an academic paper to summarize our work "[Fawkes: Protecting Personal Privacy against Unauthorized Deep Learning Models](https://www.shawnshan.com/files/publication/fawkes.pdf)" at *USENIX Security 2020*.
|
||||
|
||||
|
||||
Fawkes is a privacy protection system developed by researchers at [SANDLab](https://sandlab.cs.uchicago.edu/),
|
||||
University of Chicago. For more information about the project, please refer to our
|
||||
project [webpage](https://sandlab.cs.uchicago.edu/fawkes/). Contact us at fawkes-team@googlegroups.com.
|
||||
|
||||
We published an academic paper to summarize our
|
||||
work "[Fawkes: Protecting Personal Privacy against Unauthorized Deep Learning Models](https://www.shawnshan.com/files/publication/fawkes.pdf)"
|
||||
at *USENIX Security 2020*.
|
||||
|
||||
|
||||
Copyright
|
||||
@ -21,31 +22,30 @@ Usage
|
||||
|
||||
Options:
|
||||
|
||||
* `-m`, `--mode` : the tradeoff between privacy and perturbation size. Select from `min`, `low`, `mid`, `high`. The higher the mode is, the more perturbation will add to the image and provide stronger protection.
|
||||
* `-m`, `--mode` : the tradeoff between privacy and perturbation size. Select from `low`, `mid`, `high`. The
|
||||
higher the mode is, the more perturbation will add to the image and provide stronger protection.
|
||||
* `-d`, `--directory` : the directory with images to run protection.
|
||||
* `-g`, `--gpu` : the GPU id when using GPU for optimization.
|
||||
* `--batch-size` : number of images to run optimization together. Change to >1 only if you have extremely powerful compute power.
|
||||
* `--batch-size` : number of images to run optimization together. Change to >1 only if you have extremely powerful
|
||||
compute power.
|
||||
* `--format` : format of the output image (png or jpg).
|
||||
|
||||
when --mode is `custom`:
|
||||
* `--th` : perturbation threshold
|
||||
* `--max-step` : number of optimization steps to run
|
||||
* `--lr` : learning rate for the optimization
|
||||
* `--feature-extractor` : name of the feature extractor to use
|
||||
* `--separate_target` : whether select separate targets for each faces in the diectory.
|
||||
|
||||
### Example
|
||||
|
||||
`fawkes -d ./imgs --mode min`
|
||||
|
||||
### Tips
|
||||
- The perturbation generation takes ~60 seconds per image on a CPU machine, and it would be much faster on a GPU machine. Use `batch-size=1` on CPU and `batch-size>1` on GPUs.
|
||||
- Turn on separate target if the images in the directory belong to different people, otherwise, turn it off.
|
||||
- Run on GPU. The current Fawkes package and binary does not support GPU. To use GPU, you need to clone this, install the required packages in `setup.py`, and replace tensorflow with tensorflow-gpu. Then you can run Fawkes by `python3 fawkes/protection.py [args]`.
|
||||
|
||||
- The perturbation generation takes ~60 seconds per image on a CPU machine, and it would be much faster on a GPU
|
||||
machine. Use `batch-size=1` on CPU and `batch-size>1` on GPUs.
|
||||
- Run on GPU. The current Fawkes package and binary does not support GPU. To use GPU, you need to clone this repo, install
|
||||
the required packages in `setup.py`, and replace tensorflow with tensorflow-gpu. Then you can run Fawkes
|
||||
by `python3 fawkes/protection.py [args]`.
|
||||
|
||||
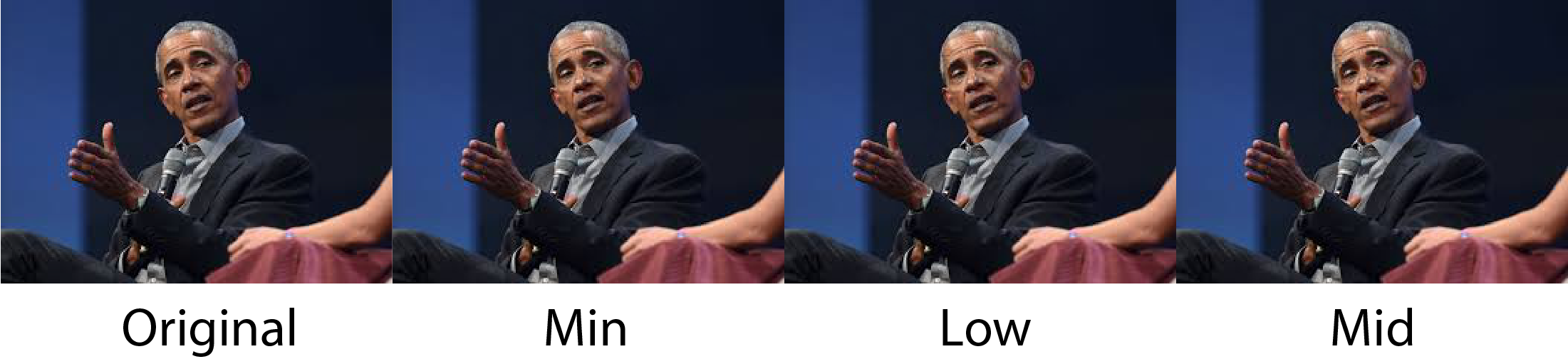
|
||||
|
||||
### How do I know my images are secure?
|
||||
|
||||
We are actively working on this. Python scripts that can test the protection effectiveness will be ready shortly.
|
||||
|
||||
Quick Installation
|
||||
@ -62,19 +62,15 @@ If you don't have root privilege, please try to install on user namespace: `pip
|
||||
|
||||
Academic Research Usage
|
||||
-----------------------
|
||||
For academic researchers, whether seeking to improve fawkes or to explore potential vunerability, please refer to the following guide to test Fawkes.
|
||||
|
||||
To protect a class in a dataset, first move the label's image to a seperate location and run Fawkes. Please use `--debug` option and set `batch-size` to a reasonable number (i.e 16, 32). If the images are already cropped and aligned, then also use the `no-align` option.
|
||||
|
||||
|
||||
|
||||
Contribute to Fawkes
|
||||
--------------------
|
||||
|
||||
If you would like to contribute to make Fawkes software better, please checkout our [project list](https://github.com/Shawn-Shan/fawkes/projects/1) which contains our TODOs. If you are confident in helping, please open a pull requests and explain the plans for your changes. We will try our best to approve asap, and once approved, you can work on it.
|
||||
For academic researchers, whether seeking to improve fawkes or to explore potential vunerability, please refer to the
|
||||
following guide to test Fawkes.
|
||||
|
||||
To protect a class in a dataset, first move the label's image to a seperate location and run Fawkes. Please
|
||||
use `--debug` option and set `batch-size` to a reasonable number (i.e 16, 32). If the images are already cropped and
|
||||
aligned, then also use the `no-align` option.
|
||||
|
||||
### Citation
|
||||
|
||||
```
|
||||
@inproceedings{shan2020fawkes,
|
||||
title={Fawkes: Protecting Personal Privacy against Unauthorized Deep Learning Models},
|
||||
|
@ -4,7 +4,8 @@ from PyQt5 import QtCore, QtWidgets
|
||||
from PyQt5.QtCore import QThread, pyqtSignal
|
||||
from PyQt5.QtWidgets import QFileDialog
|
||||
from fawkes.protection import Fawkes
|
||||
|
||||
import os
|
||||
os.environ['QT_MAC_WANTS_LAYER'] = '1'
|
||||
|
||||
class Worker(QThread):
|
||||
signal = pyqtSignal('PyQt_PyObject')
|
||||
@ -16,7 +17,7 @@ class Worker(QThread):
|
||||
|
||||
def run(self):
|
||||
if self.my_fawkes is None:
|
||||
self.my_fawkes = Fawkes("high_extract", '0', 1)
|
||||
self.my_fawkes = Fawkes("extractor_2", '0', 1)
|
||||
status = self.my_fawkes.run_protection(self.image_paths, debug=True)
|
||||
self.signal.emit(status)
|
||||
|
||||
|
@ -8,6 +8,7 @@ If you are a developer or researcher planning to customize and modify on our exi
|
||||
|
||||
#### MAC:
|
||||
|
||||
* Download the binary following this [link](http://sandlab.cs.uchicago.edu/fawkes/files/fawkes_binary.zip) and unzip the download file.
|
||||
* Create a directory and move all the images you wish to protect into that directory. Note the path to that directory (e.g. ~/Desktop/images).
|
||||
* Open [terminal](https://support.apple.com/guide/terminal/open-or-quit-terminal-apd5265185d-f365-44cb-8b09-71a064a42125/mac) and change directory to fawkes (the unzipped folder).
|
||||
* (If your MacOS is Catalina) Run `sudo spctl --master-disable` to enable running apps from unidentified developer. We are working on a solution to bypass this step.
|
||||
@ -16,12 +17,14 @@ If you are a developer or researcher planning to customize and modify on our exi
|
||||
|
||||
|
||||
#### PC:
|
||||
* Download the binary following this [link](http://sandlab.cs.uchicago.edu/fawkes/files/fawkes_binary_windows.zip) and unzip the download file.
|
||||
* Create a directory and move all the images you wish to protect into that directory. Note the path to that directory (e.g. ~/Desktop/images).
|
||||
* Open terminal(powershell or cmd) and change directory to protection (the unzipped folder).
|
||||
* Run `protection-v0.3.exe -d IMAGE_DIR_PATH` to generate cloak for images in `IMAGE_DIR_PATH`.
|
||||
* When the cloaked image is generated, it will output a `*_min_cloaked.png` image in `IMAGE_DIR_PATH`. The generation takes ~40 seconds per image depending on the hardware.
|
||||
|
||||
#### Linux:
|
||||
* Download the binary following this [link](http://sandlab.cs.uchicago.edu/fawkes/files/fawkes_binary_linux.zip) and unzip the download file.
|
||||
* Create a directory and move all the images you wish to protect into that directory. Note the path to that directory (e.g. ~/Desktop/images).
|
||||
* Open terminal and change directory to protection (the unzipped folder).
|
||||
* Run `./protection-v0.3 -d IMAGE_DIR_PATH` to generate cloak for images in `IMAGE_DIR_PATH`.
|
||||
|
@ -4,16 +4,15 @@
|
||||
# @Link : https://www.shawnshan.com/
|
||||
|
||||
|
||||
__version__ = '0.3.1'
|
||||
__version__ = '1.0.1'
|
||||
|
||||
from .detect_faces import create_mtcnn, run_detect_face
|
||||
from .differentiator import FawkesMaskGeneration
|
||||
from .protection import main, Fawkes
|
||||
from .utils import load_extractor, init_gpu, select_target_label, dump_image, reverse_process_cloaked, Faces, get_file, \
|
||||
filter_image_paths
|
||||
|
||||
__all__ = (
|
||||
'__version__', 'create_mtcnn', 'run_detect_face',
|
||||
'__version__',
|
||||
'FawkesMaskGeneration', 'load_extractor',
|
||||
'init_gpu',
|
||||
'select_target_label', 'dump_image', 'reverse_process_cloaked',
|
||||
|
@ -1,36 +1,5 @@
|
||||
"""Performs face alignment and stores face thumbnails in the output directory."""
|
||||
# MIT License
|
||||
#
|
||||
# Copyright (c) 2016 David Sandberg
|
||||
#
|
||||
# Permission is hereby granted, free of charge, to any person obtaining a copy
|
||||
# of this software and associated documentation files (the "Software"), to deal
|
||||
# in the Software without restriction, including without limitation the rights
|
||||
# to use, copy, modify, merge, publish, distribute, sublicense, and/or sell
|
||||
# copies of the Software, and to permit persons to whom the Software is
|
||||
# furnished to do so, subject to the following conditions:
|
||||
#
|
||||
# The above copyright notice and this permission notice shall be included in all
|
||||
# copies or substantial portions of the Software.
|
||||
#
|
||||
# THE SOFTWARE IS PROVIDED "AS IS", WITHOUT WARRANTY OF ANY KIND, EXPRESS OR
|
||||
# IMPLIED, INCLUDING BUT NOT LIMITED TO THE WARRANTIES OF MERCHANTABILITY,
|
||||
# FITNESS FOR A PARTICULAR PURPOSE AND NONINFRINGEMENT. IN NO EVENT SHALL THE
|
||||
# AUTHORS OR COPYRIGHT HOLDERS BE LIABLE FOR ANY CLAIM, DAMAGES OR OTHER
|
||||
# LIABILITY, WHETHER IN AN ACTION OF CONTRACT, TORT OR OTHERWISE, ARISING FROM,
|
||||
# OUT OF OR IN CONNECTION WITH THE SOFTWARE OR THE USE OR OTHER DEALINGS IN THE
|
||||
# SOFTWARE.
|
||||
|
||||
""" Tensorflow implementation of the face detection / alignment algorithm found at
|
||||
https://github.com/kpzhang93/MTCNN_face_detection_alignment
|
||||
"""
|
||||
|
||||
|
||||
import numpy as np
|
||||
from fawkes import create_mtcnn, run_detect_face
|
||||
|
||||
np_load_old = np.load
|
||||
np.load = lambda *a, **k: np_load_old(*a, allow_pickle=True, **k)
|
||||
from mtcnn import MTCNN
|
||||
|
||||
|
||||
def to_rgb(img):
|
||||
@ -39,16 +8,13 @@ def to_rgb(img):
|
||||
ret[:, :, 0] = ret[:, :, 1] = ret[:, :, 2] = img
|
||||
return ret
|
||||
|
||||
|
||||
def aligner(sess):
|
||||
pnet, rnet, onet = create_mtcnn(sess, None)
|
||||
return [pnet, rnet, onet]
|
||||
|
||||
def aligner():
|
||||
return MTCNN()
|
||||
|
||||
def align(orig_img, aligner, margin=0.8, detect_multiple_faces=True):
|
||||
pnet, rnet, onet = aligner
|
||||
minsize = 25 # minimum size of face
|
||||
threshold = [0.85, 0.85, 0.85] # three steps's threshold
|
||||
""" run MTCNN face detector """
|
||||
minsize = 20 # minimum size of face
|
||||
threshold = [0.6, 0.7, 0.7] # three steps's threshold
|
||||
factor = 0.709 # scale factor
|
||||
|
||||
if orig_img.ndim < 2:
|
||||
@ -57,40 +23,43 @@ def align(orig_img, aligner, margin=0.8, detect_multiple_faces=True):
|
||||
orig_img = to_rgb(orig_img)
|
||||
orig_img = orig_img[:, :, 0:3]
|
||||
|
||||
bounding_boxes, _ = run_detect_face(orig_img, minsize, pnet, rnet, onet, threshold, factor)
|
||||
nrof_faces = bounding_boxes.shape[0]
|
||||
bounding_boxes = aligner.detect_faces(orig_img)
|
||||
nrof_faces= len(bounding_boxes)
|
||||
|
||||
if nrof_faces > 0:
|
||||
det = bounding_boxes[:, 0:4]
|
||||
det = bounding_boxes[0]['box']
|
||||
det_arr = []
|
||||
img_size = np.asarray(orig_img.shape)[0:2]
|
||||
if nrof_faces > 1:
|
||||
margin = margin / 1.5
|
||||
if detect_multiple_faces:
|
||||
for i in range(nrof_faces):
|
||||
det_arr.append(np.squeeze(det[i]))
|
||||
det_arr.append(np.squeeze(bounding_boxes[i]['box']))
|
||||
else:
|
||||
bounding_box_size = (det[:, 2] - det[:, 0]) * (det[:, 3] - det[:, 1])
|
||||
bounding_box_size = (det[1] + det[3])
|
||||
img_center = img_size / 2
|
||||
offsets = np.vstack([(det[:, 0] + det[:, 2]) / 2 - img_center[1],
|
||||
(det[:, 1] + det[:, 3]) / 2 - img_center[0]])
|
||||
offsets = np.vstack([(det[0] + det[2]) / 2 - img_center[1],
|
||||
(det[1] + det[3]) / 2 - img_center[0]])
|
||||
offset_dist_squared = np.sum(np.power(offsets, 2.0), 0)
|
||||
index = np.argmax(bounding_box_size - offset_dist_squared * 2.0) # some extra weight on the centering
|
||||
det_arr.append(det[index, :])
|
||||
else:
|
||||
det_arr.append(np.squeeze(det))
|
||||
|
||||
cropped_arr = []
|
||||
bounding_boxes_arr = []
|
||||
for i, det in enumerate(det_arr):
|
||||
det = np.squeeze(det)
|
||||
bb = np.zeros(4, dtype=np.int32)
|
||||
side_1 = int((det[2] - det[0]) * margin)
|
||||
side_2 = int((det[3] - det[1]) * margin)
|
||||
# add in margin
|
||||
marg1 = int((det[2] - det[0]) * margin)
|
||||
marg2 = int((det[3] - det[1]) * margin)
|
||||
|
||||
bb[0] = np.maximum(det[0] - side_1 / 2, 0)
|
||||
bb[1] = np.maximum(det[1] - side_1 / 2, 0)
|
||||
bb[2] = np.minimum(det[2] + side_2 / 2, img_size[1])
|
||||
bb[3] = np.minimum(det[3] + side_2 / 2, img_size[0])
|
||||
cropped = orig_img[bb[1]:bb[3], bb[0]:bb[2], :]
|
||||
bb[0] = max(det[0] - marg1/2, 0)
|
||||
bb[1] = max(det[1] - marg2/2, 0)
|
||||
bb[2] = min(det[0] + det[2] + marg1/2, img_size[0])
|
||||
bb[3] = min(det[1] + det[3] + marg2/2, img_size[1])
|
||||
cropped = orig_img[bb[0]:bb[2], bb[1]: bb[3],:]
|
||||
cropped_arr.append(cropped)
|
||||
bounding_boxes_arr.append([bb[0], bb[1], bb[2], bb[3]])
|
||||
return cropped_arr, bounding_boxes_arr
|
||||
|
@ -1,803 +0,0 @@
|
||||
"""Performs face alignment and stores face thumbnails in the output directory."""
|
||||
# MIT License
|
||||
#
|
||||
# Copyright (c) 2016 David Sandberg
|
||||
#
|
||||
# Permission is hereby granted, free of charge, to any person obtaining a copy
|
||||
# of this software and associated documentation files (the "Software"), to deal
|
||||
# in the Software without restriction, including without limitation the rights
|
||||
# to use, copy, modify, merge, publish, distribute, sublicense, and/or sell
|
||||
# copies of the Software, and to permit persons to whom the Software is
|
||||
# furnished to do so, subject to the following conditions:
|
||||
#
|
||||
# The above copyright notice and this permission notice shall be included in all
|
||||
# copies or substantial portions of the Software.
|
||||
#
|
||||
# THE SOFTWARE IS PROVIDED "AS IS", WITHOUT WARRANTY OF ANY KIND, EXPRESS OR
|
||||
# IMPLIED, INCLUDING BUT NOT LIMITED TO THE WARRANTIES OF MERCHANTABILITY,
|
||||
# FITNESS FOR A PARTICULAR PURPOSE AND NONINFRINGEMENT. IN NO EVENT SHALL THE
|
||||
# AUTHORS OR COPYRIGHT HOLDERS BE LIABLE FOR ANY CLAIM, DAMAGES OR OTHER
|
||||
# LIABILITY, WHETHER IN AN ACTION OF CONTRACT, TORT OR OTHERWISE, ARISING FROM,
|
||||
# OUT OF OR IN CONNECTION WITH THE SOFTWARE OR THE USE OR OTHER DEALINGS IN THE
|
||||
# SOFTWARE.
|
||||
|
||||
""" Tensorflow implementation of the face detection / alignment algorithm found at
|
||||
https://github.com/kpzhang93/MTCNN_face_detection_alignment
|
||||
"""
|
||||
|
||||
import gzip
|
||||
import os
|
||||
import pickle
|
||||
|
||||
import numpy as np
|
||||
import tensorflow as tf
|
||||
from six import string_types, iteritems
|
||||
|
||||
|
||||
def layer(op):
|
||||
"""Decorator for composable network layers."""
|
||||
|
||||
def layer_decorated(self, *args, **kwargs):
|
||||
# Automatically set a name if not provided.
|
||||
name = kwargs.setdefault('name', self.get_unique_name(op.__name__))
|
||||
# Figure out the layer inputs.
|
||||
if len(self.terminals) == 0:
|
||||
raise RuntimeError('No input variables found for layer %s.' % name)
|
||||
elif len(self.terminals) == 1:
|
||||
layer_input = self.terminals[0]
|
||||
else:
|
||||
layer_input = list(self.terminals)
|
||||
# Perform the operation and get the output.
|
||||
layer_output = op(self, layer_input, *args, **kwargs)
|
||||
# Add to layer LUT.
|
||||
self.layers[name] = layer_output
|
||||
# This output is now the input for the next layer.
|
||||
self.feed(layer_output)
|
||||
# Return self for chained calls.
|
||||
return self
|
||||
|
||||
return layer_decorated
|
||||
|
||||
|
||||
class Network(object):
|
||||
|
||||
def __init__(self, inputs, trainable=True):
|
||||
# The input nodes for this network
|
||||
self.inputs = inputs
|
||||
# The current list of terminal nodes
|
||||
self.terminals = []
|
||||
# Mapping from layer names to layers
|
||||
self.layers = dict(inputs)
|
||||
# If true, the resulting variables are set as trainable
|
||||
self.trainable = trainable
|
||||
|
||||
self.setup()
|
||||
|
||||
def setup(self):
|
||||
"""Construct the network. """
|
||||
raise NotImplementedError('Must be implemented by the subclass.')
|
||||
|
||||
def load(self, data_dict, session, ignore_missing=False):
|
||||
"""Load network weights.
|
||||
data_path: The path to the numpy-serialized network weights
|
||||
session: The current TensorFlow session
|
||||
ignore_missing: If true, serialized weights for missing layers are ignored.
|
||||
"""
|
||||
|
||||
for op_name in data_dict:
|
||||
with tf.variable_scope(op_name, reuse=True):
|
||||
for param_name, data in iteritems(data_dict[op_name]):
|
||||
try:
|
||||
var = tf.get_variable(param_name)
|
||||
session.run(var.assign(data))
|
||||
except ValueError:
|
||||
if not ignore_missing:
|
||||
raise
|
||||
|
||||
def feed(self, *args):
|
||||
"""Set the input(s) for the next operation by replacing the terminal nodes.
|
||||
The arguments can be either layer names or the actual layers.
|
||||
"""
|
||||
assert len(args) != 0
|
||||
self.terminals = []
|
||||
for fed_layer in args:
|
||||
if isinstance(fed_layer, string_types):
|
||||
try:
|
||||
fed_layer = self.layers[fed_layer]
|
||||
except KeyError:
|
||||
raise KeyError('Unknown layer name fed: %s' % fed_layer)
|
||||
self.terminals.append(fed_layer)
|
||||
return self
|
||||
|
||||
def get_output(self):
|
||||
"""Returns the current network output."""
|
||||
return self.terminals[-1]
|
||||
|
||||
def get_unique_name(self, prefix):
|
||||
"""Returns an index-suffixed unique name for the given prefix.
|
||||
This is used for auto-generating layer names based on the type-prefix.
|
||||
"""
|
||||
ident = sum(t.startswith(prefix) for t, _ in self.layers.items()) + 1
|
||||
return '%s_%d' % (prefix, ident)
|
||||
|
||||
def make_var(self, name, shape):
|
||||
"""Creates a new TensorFlow variable."""
|
||||
return tf.get_variable(name, shape, trainable=self.trainable)
|
||||
|
||||
def validate_padding(self, padding):
|
||||
"""Verifies that the padding is one of the supported ones."""
|
||||
assert padding in ('SAME', 'VALID')
|
||||
|
||||
@layer
|
||||
def conv(self,
|
||||
inp,
|
||||
k_h,
|
||||
k_w,
|
||||
c_o,
|
||||
s_h,
|
||||
s_w,
|
||||
name,
|
||||
relu=True,
|
||||
padding='SAME',
|
||||
group=1,
|
||||
biased=True):
|
||||
# Verify that the padding is acceptable
|
||||
self.validate_padding(padding)
|
||||
# Get the number of channels in the input
|
||||
c_i = int(inp.get_shape()[-1])
|
||||
# Verify that the grouping parameter is valid
|
||||
assert c_i % group == 0
|
||||
assert c_o % group == 0
|
||||
# Convolution for a given input and kernel
|
||||
convolve = lambda i, k: tf.nn.conv2d(i, k, [1, s_h, s_w, 1], padding=padding)
|
||||
with tf.variable_scope(name) as scope:
|
||||
kernel = self.make_var('weights', shape=[k_h, k_w, c_i // group, c_o])
|
||||
# This is the common-case. Convolve the input without any further complications.
|
||||
output = convolve(inp, kernel)
|
||||
# Add the biases
|
||||
if biased:
|
||||
biases = self.make_var('biases', [c_o])
|
||||
output = tf.nn.bias_add(output, biases)
|
||||
if relu:
|
||||
# ReLU non-linearity
|
||||
output = tf.nn.relu(output, name=scope.name)
|
||||
return output
|
||||
|
||||
@layer
|
||||
def prelu(self, inp, name):
|
||||
with tf.variable_scope(name):
|
||||
i = int(inp.get_shape()[-1])
|
||||
alpha = self.make_var('alpha', shape=(i,))
|
||||
output = tf.nn.relu(inp) + tf.multiply(alpha, -tf.nn.relu(-inp))
|
||||
return output
|
||||
|
||||
@layer
|
||||
def max_pool(self, inp, k_h, k_w, s_h, s_w, name, padding='SAME'):
|
||||
self.validate_padding(padding)
|
||||
return tf.nn.max_pool(inp,
|
||||
ksize=[1, k_h, k_w, 1],
|
||||
strides=[1, s_h, s_w, 1],
|
||||
padding=padding,
|
||||
name=name)
|
||||
|
||||
@layer
|
||||
def fc(self, inp, num_out, name, relu=True):
|
||||
with tf.variable_scope(name):
|
||||
input_shape = inp.get_shape()
|
||||
if input_shape.ndims == 4:
|
||||
# The input is spatial. Vectorize it first.
|
||||
dim = 1
|
||||
for d in input_shape[1:].as_list():
|
||||
dim *= int(d)
|
||||
feed_in = tf.reshape(inp, [-1, dim])
|
||||
else:
|
||||
feed_in, dim = (inp, input_shape[-1].value)
|
||||
weights = self.make_var('weights', shape=[dim, num_out])
|
||||
biases = self.make_var('biases', [num_out])
|
||||
op = tf.nn.relu_layer if relu else tf.nn.xw_plus_b
|
||||
fc = op(feed_in, weights, biases, name=name)
|
||||
return fc
|
||||
|
||||
"""
|
||||
Multi dimensional softmax,
|
||||
refer to https://github.com/tensorflow/tensorflow/issues/210
|
||||
compute softmax along the dimension of target
|
||||
the native softmax only supports batch_size x dimension
|
||||
"""
|
||||
|
||||
@layer
|
||||
def softmax(self, target, axis, name=None):
|
||||
max_axis = tf.reduce_max(target, axis, keepdims=True)
|
||||
target_exp = tf.exp(target - max_axis)
|
||||
normalize = tf.reduce_sum(target_exp, axis, keepdims=True)
|
||||
softmax = tf.div(target_exp, normalize, name)
|
||||
return softmax
|
||||
|
||||
|
||||
class PNet(Network):
|
||||
def setup(self):
|
||||
(self.feed('data') # pylint: disable=no-value-for-parameter, no-member
|
||||
.conv(3, 3, 10, 1, 1, padding='VALID', relu=False, name='conv1')
|
||||
.prelu(name='PReLU1')
|
||||
.max_pool(2, 2, 2, 2, name='pool1')
|
||||
.conv(3, 3, 16, 1, 1, padding='VALID', relu=False, name='conv2')
|
||||
.prelu(name='PReLU2')
|
||||
.conv(3, 3, 32, 1, 1, padding='VALID', relu=False, name='conv3')
|
||||
.prelu(name='PReLU3')
|
||||
.conv(1, 1, 2, 1, 1, relu=False, name='conv4-1')
|
||||
.softmax(3, name='prob1'))
|
||||
|
||||
(self.feed('PReLU3') # pylint: disable=no-value-for-parameter
|
||||
.conv(1, 1, 4, 1, 1, relu=False, name='conv4-2'))
|
||||
|
||||
|
||||
class RNet(Network):
|
||||
def setup(self):
|
||||
(self.feed('data') # pylint: disable=no-value-for-parameter, no-member
|
||||
.conv(3, 3, 28, 1, 1, padding='VALID', relu=False, name='conv1')
|
||||
.prelu(name='prelu1')
|
||||
.max_pool(3, 3, 2, 2, name='pool1')
|
||||
.conv(3, 3, 48, 1, 1, padding='VALID', relu=False, name='conv2')
|
||||
.prelu(name='prelu2')
|
||||
.max_pool(3, 3, 2, 2, padding='VALID', name='pool2')
|
||||
.conv(2, 2, 64, 1, 1, padding='VALID', relu=False, name='conv3')
|
||||
.prelu(name='prelu3')
|
||||
.fc(128, relu=False, name='conv4')
|
||||
.prelu(name='prelu4')
|
||||
.fc(2, relu=False, name='conv5-1')
|
||||
.softmax(1, name='prob1'))
|
||||
|
||||
(self.feed('prelu4') # pylint: disable=no-value-for-parameter
|
||||
.fc(4, relu=False, name='conv5-2'))
|
||||
|
||||
|
||||
class ONet(Network):
|
||||
def setup(self):
|
||||
(self.feed('data') # pylint: disable=no-value-for-parameter, no-member
|
||||
.conv(3, 3, 32, 1, 1, padding='VALID', relu=False, name='conv1')
|
||||
.prelu(name='prelu1')
|
||||
.max_pool(3, 3, 2, 2, name='pool1')
|
||||
.conv(3, 3, 64, 1, 1, padding='VALID', relu=False, name='conv2')
|
||||
.prelu(name='prelu2')
|
||||
.max_pool(3, 3, 2, 2, padding='VALID', name='pool2')
|
||||
.conv(3, 3, 64, 1, 1, padding='VALID', relu=False, name='conv3')
|
||||
.prelu(name='prelu3')
|
||||
.max_pool(2, 2, 2, 2, name='pool3')
|
||||
.conv(2, 2, 128, 1, 1, padding='VALID', relu=False, name='conv4')
|
||||
.prelu(name='prelu4')
|
||||
.fc(256, relu=False, name='conv5')
|
||||
.prelu(name='prelu5')
|
||||
.fc(2, relu=False, name='conv6-1')
|
||||
.softmax(1, name='prob1'))
|
||||
|
||||
(self.feed('prelu5') # pylint: disable=no-value-for-parameter
|
||||
.fc(4, relu=False, name='conv6-2'))
|
||||
|
||||
(self.feed('prelu5') # pylint: disable=no-value-for-parameter
|
||||
.fc(10, relu=False, name='conv6-3'))
|
||||
|
||||
|
||||
def create_mtcnn(sess, model_path):
|
||||
model_dir = os.path.join(os.path.expanduser('~'), '.fawkes')
|
||||
os.makedirs(model_dir, exist_ok=True)
|
||||
|
||||
fp = gzip.open(os.path.join(model_dir, "mtcnn.p.gz"), 'rb')
|
||||
dnet_weights = pickle.load(fp)
|
||||
fp.close()
|
||||
|
||||
with tf.variable_scope('pnet'):
|
||||
data = tf.placeholder(tf.float32, (None, None, None, 3), 'input')
|
||||
pnet = PNet({'data': data})
|
||||
|
||||
# data_dict = np.load(data_path, encoding='latin1').item() # pylint: disable=no-member
|
||||
pnet.load(dnet_weights[0], sess)
|
||||
with tf.variable_scope('rnet'):
|
||||
data = tf.placeholder(tf.float32, (None, 24, 24, 3), 'input')
|
||||
rnet = RNet({'data': data})
|
||||
rnet.load(dnet_weights[1], sess)
|
||||
with tf.variable_scope('onet'):
|
||||
data = tf.placeholder(tf.float32, (None, 48, 48, 3), 'input')
|
||||
onet = ONet({'data': data})
|
||||
onet.load(dnet_weights[2], sess)
|
||||
|
||||
pnet_fun = lambda img: sess.run(('pnet/conv4-2/BiasAdd:0', 'pnet/prob1:0'), feed_dict={'pnet/input:0': img})
|
||||
rnet_fun = lambda img: sess.run(('rnet/conv5-2/conv5-2:0', 'rnet/prob1:0'), feed_dict={'rnet/input:0': img})
|
||||
onet_fun = lambda img: sess.run(('onet/conv6-2/conv6-2:0', 'onet/conv6-3/conv6-3:0', 'onet/prob1:0'),
|
||||
feed_dict={'onet/input:0': img})
|
||||
return pnet_fun, rnet_fun, onet_fun
|
||||
|
||||
|
||||
def run_detect_face(img, minsize, pnet, rnet, onet, threshold, factor):
|
||||
"""Detects faces in an image, and returns bounding boxes and points for them.
|
||||
img: input image
|
||||
minsize: minimum faces' size
|
||||
pnet, rnet, onet: caffemodel
|
||||
threshold: threshold=[th1, th2, th3], th1-3 are three steps's threshold
|
||||
factor: the factor used to create a scaling pyramid of face sizes to detect in the image.
|
||||
"""
|
||||
factor_count = 0
|
||||
total_boxes = np.empty((0, 9))
|
||||
points = np.empty(0)
|
||||
h = img.shape[0]
|
||||
w = img.shape[1]
|
||||
minl = np.amin([h, w])
|
||||
m = 12.0 / minsize
|
||||
minl = minl * m
|
||||
# create scale pyramid
|
||||
scales = []
|
||||
while minl >= 12:
|
||||
scales += [m * np.power(factor, factor_count)]
|
||||
minl = minl * factor
|
||||
factor_count += 1
|
||||
|
||||
# first stage
|
||||
for scale in scales:
|
||||
hs = int(np.ceil(h * scale))
|
||||
ws = int(np.ceil(w * scale))
|
||||
im_data = imresample(img, (hs, ws))
|
||||
im_data = (im_data - 127.5) * 0.0078125
|
||||
img_x = np.expand_dims(im_data, 0)
|
||||
img_y = np.transpose(img_x, (0, 2, 1, 3))
|
||||
out = pnet(img_y)
|
||||
out0 = np.transpose(out[0], (0, 2, 1, 3))
|
||||
out1 = np.transpose(out[1], (0, 2, 1, 3))
|
||||
|
||||
boxes, _ = generateBoundingBox(out1[0, :, :, 1].copy(), out0[0, :, :, :].copy(), scale, threshold[0])
|
||||
|
||||
# inter-scale nms
|
||||
pick = nms(boxes.copy(), 0.5, 'Union')
|
||||
if boxes.size > 0 and pick.size > 0:
|
||||
boxes = boxes[pick, :]
|
||||
total_boxes = np.append(total_boxes, boxes, axis=0)
|
||||
|
||||
numbox = total_boxes.shape[0]
|
||||
if numbox > 0:
|
||||
pick = nms(total_boxes.copy(), 0.7, 'Union')
|
||||
total_boxes = total_boxes[pick, :]
|
||||
regw = total_boxes[:, 2] - total_boxes[:, 0]
|
||||
regh = total_boxes[:, 3] - total_boxes[:, 1]
|
||||
qq1 = total_boxes[:, 0] + total_boxes[:, 5] * regw
|
||||
qq2 = total_boxes[:, 1] + total_boxes[:, 6] * regh
|
||||
qq3 = total_boxes[:, 2] + total_boxes[:, 7] * regw
|
||||
qq4 = total_boxes[:, 3] + total_boxes[:, 8] * regh
|
||||
total_boxes = np.transpose(np.vstack([qq1, qq2, qq3, qq4, total_boxes[:, 4]]))
|
||||
total_boxes = rerec(total_boxes.copy())
|
||||
total_boxes[:, 0:4] = np.fix(total_boxes[:, 0:4]).astype(np.int32)
|
||||
dy, edy, dx, edx, y, ey, x, ex, tmpw, tmph = pad(total_boxes.copy(), w, h)
|
||||
|
||||
numbox = total_boxes.shape[0]
|
||||
if numbox > 0:
|
||||
# second stage
|
||||
tempimg = np.zeros((24, 24, 3, numbox))
|
||||
for k in range(0, numbox):
|
||||
tmp = np.zeros((int(tmph[k]), int(tmpw[k]), 3))
|
||||
# try:
|
||||
tmp[dy[k] - 1:edy[k], dx[k] - 1:edx[k], :] = img[y[k] - 1:ey[k], x[k] - 1:ex[k], :]
|
||||
# except ValueError:
|
||||
# continue
|
||||
if tmp.shape[0] > 0 and tmp.shape[1] > 0 or tmp.shape[0] == 0 and tmp.shape[1] == 0:
|
||||
tempimg[:, :, :, k] = imresample(tmp, (24, 24))
|
||||
else:
|
||||
return np.empty()
|
||||
|
||||
tempimg = (tempimg - 127.5) * 0.0078125
|
||||
tempimg1 = np.transpose(tempimg, (3, 1, 0, 2))
|
||||
out = rnet(tempimg1)
|
||||
out0 = np.transpose(out[0])
|
||||
out1 = np.transpose(out[1])
|
||||
score = out1[1, :]
|
||||
ipass = np.where(score > threshold[1])
|
||||
total_boxes = np.hstack([total_boxes[ipass[0], 0:4].copy(), np.expand_dims(score[ipass].copy(), 1)])
|
||||
mv = out0[:, ipass[0]]
|
||||
if total_boxes.shape[0] > 0:
|
||||
pick = nms(total_boxes, 0.7, 'Union')
|
||||
total_boxes = total_boxes[pick, :]
|
||||
total_boxes = bbreg(total_boxes.copy(), np.transpose(mv[:, pick]))
|
||||
total_boxes = rerec(total_boxes.copy())
|
||||
|
||||
numbox = total_boxes.shape[0]
|
||||
if numbox > 0:
|
||||
# third stage
|
||||
total_boxes = np.fix(total_boxes).astype(np.int32)
|
||||
dy, edy, dx, edx, y, ey, x, ex, tmpw, tmph = pad(total_boxes.copy(), w, h)
|
||||
tempimg = np.zeros((48, 48, 3, numbox))
|
||||
for k in range(0, numbox):
|
||||
tmp = np.zeros((int(tmph[k]), int(tmpw[k]), 3))
|
||||
tmp[dy[k] - 1:edy[k], dx[k] - 1:edx[k], :] = img[y[k] - 1:ey[k], x[k] - 1:ex[k], :]
|
||||
if tmp.shape[0] > 0 and tmp.shape[1] > 0 or tmp.shape[0] == 0 and tmp.shape[1] == 0:
|
||||
tempimg[:, :, :, k] = imresample(tmp, (48, 48))
|
||||
else:
|
||||
return np.empty()
|
||||
tempimg = (tempimg - 127.5) * 0.0078125
|
||||
tempimg1 = np.transpose(tempimg, (3, 1, 0, 2))
|
||||
out = onet(tempimg1)
|
||||
out0 = np.transpose(out[0])
|
||||
out1 = np.transpose(out[1])
|
||||
out2 = np.transpose(out[2])
|
||||
score = out2[1, :]
|
||||
points = out1
|
||||
ipass = np.where(score > threshold[2])
|
||||
points = points[:, ipass[0]]
|
||||
total_boxes = np.hstack([total_boxes[ipass[0], 0:4].copy(), np.expand_dims(score[ipass].copy(), 1)])
|
||||
mv = out0[:, ipass[0]]
|
||||
|
||||
w = total_boxes[:, 2] - total_boxes[:, 0] + 1
|
||||
h = total_boxes[:, 3] - total_boxes[:, 1] + 1
|
||||
points[0:5, :] = np.tile(w, (5, 1)) * points[0:5, :] + np.tile(total_boxes[:, 0], (5, 1)) - 1
|
||||
points[5:10, :] = np.tile(h, (5, 1)) * points[5:10, :] + np.tile(total_boxes[:, 1], (5, 1)) - 1
|
||||
if total_boxes.shape[0] > 0:
|
||||
total_boxes = bbreg(total_boxes.copy(), np.transpose(mv))
|
||||
pick = nms(total_boxes.copy(), 0.7, 'Min')
|
||||
total_boxes = total_boxes[pick, :]
|
||||
points = points[:, pick]
|
||||
|
||||
return total_boxes, points
|
||||
|
||||
|
||||
def bulk_detect_face(images, detection_window_size_ratio, pnet, rnet, onet, threshold, factor):
|
||||
"""Detects faces in a list of images
|
||||
images: list containing input images
|
||||
detection_window_size_ratio: ratio of minimum face size to smallest image dimension
|
||||
pnet, rnet, onet: caffemodel
|
||||
threshold: threshold=[th1 th2 th3], th1-3 are three steps's threshold [0-1]
|
||||
factor: the factor used to create a scaling pyramid of face sizes to detect in the image.
|
||||
"""
|
||||
all_scales = [None] * len(images)
|
||||
images_with_boxes = [None] * len(images)
|
||||
|
||||
for i in range(len(images)):
|
||||
images_with_boxes[i] = {'total_boxes': np.empty((0, 9))}
|
||||
|
||||
# create scale pyramid
|
||||
for index, img in enumerate(images):
|
||||
all_scales[index] = []
|
||||
h = img.shape[0]
|
||||
w = img.shape[1]
|
||||
minsize = int(detection_window_size_ratio * np.minimum(w, h))
|
||||
factor_count = 0
|
||||
minl = np.amin([h, w])
|
||||
if minsize <= 12:
|
||||
minsize = 12
|
||||
|
||||
m = 12.0 / minsize
|
||||
minl = minl * m
|
||||
while minl >= 12:
|
||||
all_scales[index].append(m * np.power(factor, factor_count))
|
||||
minl = minl * factor
|
||||
factor_count += 1
|
||||
|
||||
# # # # # # # # # # # # #
|
||||
# first stage - fast proposal network (pnet) to obtain face candidates
|
||||
# # # # # # # # # # # # #
|
||||
|
||||
images_obj_per_resolution = {}
|
||||
|
||||
# TODO: use some type of rounding to number module 8 to increase probability that pyramid images will have the same resolution across input images
|
||||
|
||||
for index, scales in enumerate(all_scales):
|
||||
h = images[index].shape[0]
|
||||
w = images[index].shape[1]
|
||||
|
||||
for scale in scales:
|
||||
hs = int(np.ceil(h * scale))
|
||||
ws = int(np.ceil(w * scale))
|
||||
|
||||
if (ws, hs) not in images_obj_per_resolution:
|
||||
images_obj_per_resolution[(ws, hs)] = []
|
||||
|
||||
im_data = imresample(images[index], (hs, ws))
|
||||
im_data = (im_data - 127.5) * 0.0078125
|
||||
img_y = np.transpose(im_data, (1, 0, 2)) # caffe uses different dimensions ordering
|
||||
images_obj_per_resolution[(ws, hs)].append({'scale': scale, 'image': img_y, 'index': index})
|
||||
|
||||
for resolution in images_obj_per_resolution:
|
||||
images_per_resolution = [i['image'] for i in images_obj_per_resolution[resolution]]
|
||||
outs = pnet(images_per_resolution)
|
||||
|
||||
for index in range(len(outs[0])):
|
||||
scale = images_obj_per_resolution[resolution][index]['scale']
|
||||
image_index = images_obj_per_resolution[resolution][index]['index']
|
||||
out0 = np.transpose(outs[0][index], (1, 0, 2))
|
||||
out1 = np.transpose(outs[1][index], (1, 0, 2))
|
||||
|
||||
boxes, _ = generateBoundingBox(out1[:, :, 1].copy(), out0[:, :, :].copy(), scale, threshold[0])
|
||||
|
||||
# inter-scale nms
|
||||
pick = nms(boxes.copy(), 0.5, 'Union')
|
||||
if boxes.size > 0 and pick.size > 0:
|
||||
boxes = boxes[pick, :]
|
||||
images_with_boxes[image_index]['total_boxes'] = np.append(images_with_boxes[image_index]['total_boxes'],
|
||||
boxes,
|
||||
axis=0)
|
||||
|
||||
for index, image_obj in enumerate(images_with_boxes):
|
||||
numbox = image_obj['total_boxes'].shape[0]
|
||||
if numbox > 0:
|
||||
h = images[index].shape[0]
|
||||
w = images[index].shape[1]
|
||||
pick = nms(image_obj['total_boxes'].copy(), 0.7, 'Union')
|
||||
image_obj['total_boxes'] = image_obj['total_boxes'][pick, :]
|
||||
regw = image_obj['total_boxes'][:, 2] - image_obj['total_boxes'][:, 0]
|
||||
regh = image_obj['total_boxes'][:, 3] - image_obj['total_boxes'][:, 1]
|
||||
qq1 = image_obj['total_boxes'][:, 0] + image_obj['total_boxes'][:, 5] * regw
|
||||
qq2 = image_obj['total_boxes'][:, 1] + image_obj['total_boxes'][:, 6] * regh
|
||||
qq3 = image_obj['total_boxes'][:, 2] + image_obj['total_boxes'][:, 7] * regw
|
||||
qq4 = image_obj['total_boxes'][:, 3] + image_obj['total_boxes'][:, 8] * regh
|
||||
image_obj['total_boxes'] = np.transpose(np.vstack([qq1, qq2, qq3, qq4, image_obj['total_boxes'][:, 4]]))
|
||||
image_obj['total_boxes'] = rerec(image_obj['total_boxes'].copy())
|
||||
image_obj['total_boxes'][:, 0:4] = np.fix(image_obj['total_boxes'][:, 0:4]).astype(np.int32)
|
||||
dy, edy, dx, edx, y, ey, x, ex, tmpw, tmph = pad(image_obj['total_boxes'].copy(), w, h)
|
||||
|
||||
numbox = image_obj['total_boxes'].shape[0]
|
||||
tempimg = np.zeros((24, 24, 3, numbox))
|
||||
|
||||
if numbox > 0:
|
||||
for k in range(0, numbox):
|
||||
tmp = np.zeros((int(tmph[k]), int(tmpw[k]), 3))
|
||||
tmp[dy[k] - 1:edy[k], dx[k] - 1:edx[k], :] = images[index][y[k] - 1:ey[k], x[k] - 1:ex[k], :]
|
||||
if tmp.shape[0] > 0 and tmp.shape[1] > 0 or tmp.shape[0] == 0 and tmp.shape[1] == 0:
|
||||
tempimg[:, :, :, k] = imresample(tmp, (24, 24))
|
||||
else:
|
||||
return np.empty()
|
||||
|
||||
tempimg = (tempimg - 127.5) * 0.0078125
|
||||
image_obj['rnet_input'] = np.transpose(tempimg, (3, 1, 0, 2))
|
||||
|
||||
# # # # # # # # # # # # #
|
||||
# second stage - refinement of face candidates with rnet
|
||||
# # # # # # # # # # # # #
|
||||
|
||||
bulk_rnet_input = np.empty((0, 24, 24, 3))
|
||||
for index, image_obj in enumerate(images_with_boxes):
|
||||
if 'rnet_input' in image_obj:
|
||||
bulk_rnet_input = np.append(bulk_rnet_input, image_obj['rnet_input'], axis=0)
|
||||
|
||||
out = rnet(bulk_rnet_input)
|
||||
out0 = np.transpose(out[0])
|
||||
out1 = np.transpose(out[1])
|
||||
score = out1[1, :]
|
||||
|
||||
i = 0
|
||||
for index, image_obj in enumerate(images_with_boxes):
|
||||
if 'rnet_input' not in image_obj:
|
||||
continue
|
||||
|
||||
rnet_input_count = image_obj['rnet_input'].shape[0]
|
||||
score_per_image = score[i:i + rnet_input_count]
|
||||
out0_per_image = out0[:, i:i + rnet_input_count]
|
||||
|
||||
ipass = np.where(score_per_image > threshold[1])
|
||||
image_obj['total_boxes'] = np.hstack([image_obj['total_boxes'][ipass[0], 0:4].copy(),
|
||||
np.expand_dims(score_per_image[ipass].copy(), 1)])
|
||||
|
||||
mv = out0_per_image[:, ipass[0]]
|
||||
|
||||
if image_obj['total_boxes'].shape[0] > 0:
|
||||
h = images[index].shape[0]
|
||||
w = images[index].shape[1]
|
||||
pick = nms(image_obj['total_boxes'], 0.7, 'Union')
|
||||
image_obj['total_boxes'] = image_obj['total_boxes'][pick, :]
|
||||
image_obj['total_boxes'] = bbreg(image_obj['total_boxes'].copy(), np.transpose(mv[:, pick]))
|
||||
image_obj['total_boxes'] = rerec(image_obj['total_boxes'].copy())
|
||||
|
||||
numbox = image_obj['total_boxes'].shape[0]
|
||||
|
||||
if numbox > 0:
|
||||
tempimg = np.zeros((48, 48, 3, numbox))
|
||||
image_obj['total_boxes'] = np.fix(image_obj['total_boxes']).astype(np.int32)
|
||||
dy, edy, dx, edx, y, ey, x, ex, tmpw, tmph = pad(image_obj['total_boxes'].copy(), w, h)
|
||||
|
||||
for k in range(0, numbox):
|
||||
tmp = np.zeros((int(tmph[k]), int(tmpw[k]), 3))
|
||||
tmp[dy[k] - 1:edy[k], dx[k] - 1:edx[k], :] = images[index][y[k] - 1:ey[k], x[k] - 1:ex[k], :]
|
||||
if tmp.shape[0] > 0 and tmp.shape[1] > 0 or tmp.shape[0] == 0 and tmp.shape[1] == 0:
|
||||
tempimg[:, :, :, k] = imresample(tmp, (48, 48))
|
||||
else:
|
||||
return np.empty()
|
||||
tempimg = (tempimg - 127.5) * 0.0078125
|
||||
image_obj['onet_input'] = np.transpose(tempimg, (3, 1, 0, 2))
|
||||
|
||||
i += rnet_input_count
|
||||
|
||||
# # # # # # # # # # # # #
|
||||
# third stage - further refinement and facial landmarks positions with onet
|
||||
# # # # # # # # # # # # #
|
||||
|
||||
bulk_onet_input = np.empty((0, 48, 48, 3))
|
||||
for index, image_obj in enumerate(images_with_boxes):
|
||||
if 'onet_input' in image_obj:
|
||||
bulk_onet_input = np.append(bulk_onet_input, image_obj['onet_input'], axis=0)
|
||||
|
||||
out = onet(bulk_onet_input)
|
||||
|
||||
out0 = np.transpose(out[0])
|
||||
out1 = np.transpose(out[1])
|
||||
out2 = np.transpose(out[2])
|
||||
score = out2[1, :]
|
||||
points = out1
|
||||
|
||||
i = 0
|
||||
ret = []
|
||||
for index, image_obj in enumerate(images_with_boxes):
|
||||
if 'onet_input' not in image_obj:
|
||||
ret.append(None)
|
||||
continue
|
||||
|
||||
onet_input_count = image_obj['onet_input'].shape[0]
|
||||
|
||||
out0_per_image = out0[:, i:i + onet_input_count]
|
||||
score_per_image = score[i:i + onet_input_count]
|
||||
points_per_image = points[:, i:i + onet_input_count]
|
||||
|
||||
ipass = np.where(score_per_image > threshold[2])
|
||||
points_per_image = points_per_image[:, ipass[0]]
|
||||
|
||||
image_obj['total_boxes'] = np.hstack([image_obj['total_boxes'][ipass[0], 0:4].copy(),
|
||||
np.expand_dims(score_per_image[ipass].copy(), 1)])
|
||||
mv = out0_per_image[:, ipass[0]]
|
||||
|
||||
w = image_obj['total_boxes'][:, 2] - image_obj['total_boxes'][:, 0] + 1
|
||||
h = image_obj['total_boxes'][:, 3] - image_obj['total_boxes'][:, 1] + 1
|
||||
points_per_image[0:5, :] = np.tile(w, (5, 1)) * points_per_image[0:5, :] + np.tile(
|
||||
image_obj['total_boxes'][:, 0], (5, 1)) - 1
|
||||
points_per_image[5:10, :] = np.tile(h, (5, 1)) * points_per_image[5:10, :] + np.tile(
|
||||
image_obj['total_boxes'][:, 1], (5, 1)) - 1
|
||||
|
||||
if image_obj['total_boxes'].shape[0] > 0:
|
||||
image_obj['total_boxes'] = bbreg(image_obj['total_boxes'].copy(), np.transpose(mv))
|
||||
pick = nms(image_obj['total_boxes'].copy(), 0.7, 'Min')
|
||||
image_obj['total_boxes'] = image_obj['total_boxes'][pick, :]
|
||||
points_per_image = points_per_image[:, pick]
|
||||
|
||||
ret.append((image_obj['total_boxes'], points_per_image))
|
||||
else:
|
||||
ret.append(None)
|
||||
|
||||
i += onet_input_count
|
||||
|
||||
return ret
|
||||
|
||||
|
||||
# function [boundingbox] = bbreg(boundingbox,reg)
|
||||
def bbreg(boundingbox, reg):
|
||||
"""Calibrate bounding boxes"""
|
||||
if reg.shape[1] == 1:
|
||||
reg = np.reshape(reg, (reg.shape[2], reg.shape[3]))
|
||||
|
||||
w = boundingbox[:, 2] - boundingbox[:, 0] + 1
|
||||
h = boundingbox[:, 3] - boundingbox[:, 1] + 1
|
||||
b1 = boundingbox[:, 0] + reg[:, 0] * w
|
||||
b2 = boundingbox[:, 1] + reg[:, 1] * h
|
||||
b3 = boundingbox[:, 2] + reg[:, 2] * w
|
||||
b4 = boundingbox[:, 3] + reg[:, 3] * h
|
||||
boundingbox[:, 0:4] = np.transpose(np.vstack([b1, b2, b3, b4]))
|
||||
return boundingbox
|
||||
|
||||
|
||||
def generateBoundingBox(imap, reg, scale, t):
|
||||
"""Use heatmap to generate bounding boxes"""
|
||||
stride = 2
|
||||
cellsize = 12
|
||||
|
||||
imap = np.transpose(imap)
|
||||
dx1 = np.transpose(reg[:, :, 0])
|
||||
dy1 = np.transpose(reg[:, :, 1])
|
||||
dx2 = np.transpose(reg[:, :, 2])
|
||||
dy2 = np.transpose(reg[:, :, 3])
|
||||
y, x = np.where(imap >= t)
|
||||
if y.shape[0] == 1:
|
||||
dx1 = np.flipud(dx1)
|
||||
dy1 = np.flipud(dy1)
|
||||
dx2 = np.flipud(dx2)
|
||||
dy2 = np.flipud(dy2)
|
||||
score = imap[(y, x)]
|
||||
reg = np.transpose(np.vstack([dx1[(y, x)], dy1[(y, x)], dx2[(y, x)], dy2[(y, x)]]))
|
||||
if reg.size == 0:
|
||||
reg = np.empty((0, 3))
|
||||
bb = np.transpose(np.vstack([y, x]))
|
||||
q1 = np.fix((stride * bb + 1) / scale)
|
||||
q2 = np.fix((stride * bb + cellsize - 1 + 1) / scale)
|
||||
boundingbox = np.hstack([q1, q2, np.expand_dims(score, 1), reg])
|
||||
return boundingbox, reg
|
||||
|
||||
|
||||
# function pick = nms(boxes,threshold,type)
|
||||
def nms(boxes, threshold, method):
|
||||
if boxes.size == 0:
|
||||
return np.empty((0, 3))
|
||||
x1 = boxes[:, 0]
|
||||
y1 = boxes[:, 1]
|
||||
x2 = boxes[:, 2]
|
||||
y2 = boxes[:, 3]
|
||||
s = boxes[:, 4]
|
||||
area = (x2 - x1 + 1) * (y2 - y1 + 1)
|
||||
I = np.argsort(s)
|
||||
pick = np.zeros_like(s, dtype=np.int16)
|
||||
counter = 0
|
||||
while I.size > 0:
|
||||
i = I[-1]
|
||||
pick[counter] = i
|
||||
counter += 1
|
||||
idx = I[0:-1]
|
||||
xx1 = np.maximum(x1[i], x1[idx])
|
||||
yy1 = np.maximum(y1[i], y1[idx])
|
||||
xx2 = np.minimum(x2[i], x2[idx])
|
||||
yy2 = np.minimum(y2[i], y2[idx])
|
||||
w = np.maximum(0.0, xx2 - xx1 + 1)
|
||||
h = np.maximum(0.0, yy2 - yy1 + 1)
|
||||
inter = w * h
|
||||
if method is 'Min':
|
||||
o = inter / np.minimum(area[i], area[idx])
|
||||
else:
|
||||
o = inter / (area[i] + area[idx] - inter)
|
||||
I = I[np.where(o <= threshold)]
|
||||
pick = pick[0:counter]
|
||||
return pick
|
||||
|
||||
|
||||
# function [dy edy dx edx y ey x ex tmpw tmph] = pad(total_boxes,w,h)
|
||||
def pad(total_boxes, w, h):
|
||||
"""Compute the padding coordinates (pad the bounding boxes to square)"""
|
||||
tmpw = (total_boxes[:, 2] - total_boxes[:, 0] + 1).astype(np.int32)
|
||||
tmph = (total_boxes[:, 3] - total_boxes[:, 1] + 1).astype(np.int32)
|
||||
numbox = total_boxes.shape[0]
|
||||
|
||||
dx = np.ones((numbox), dtype=np.int32)
|
||||
dy = np.ones((numbox), dtype=np.int32)
|
||||
edx = tmpw.copy().astype(np.int32)
|
||||
edy = tmph.copy().astype(np.int32)
|
||||
|
||||
x = total_boxes[:, 0].copy().astype(np.int32)
|
||||
y = total_boxes[:, 1].copy().astype(np.int32)
|
||||
ex = total_boxes[:, 2].copy().astype(np.int32)
|
||||
ey = total_boxes[:, 3].copy().astype(np.int32)
|
||||
|
||||
tmp = np.where(ex > w)
|
||||
edx.flat[tmp] = np.expand_dims(-ex[tmp] + w + tmpw[tmp], 1)
|
||||
ex[tmp] = w
|
||||
|
||||
tmp = np.where(ey > h)
|
||||
edy.flat[tmp] = np.expand_dims(-ey[tmp] + h + tmph[tmp], 1)
|
||||
ey[tmp] = h
|
||||
|
||||
tmp = np.where(x < 1)
|
||||
dx.flat[tmp] = np.expand_dims(2 - x[tmp], 1)
|
||||
x[tmp] = 1
|
||||
|
||||
tmp = np.where(y < 1)
|
||||
dy.flat[tmp] = np.expand_dims(2 - y[tmp], 1)
|
||||
y[tmp] = 1
|
||||
|
||||
return dy, edy, dx, edx, y, ey, x, ex, tmpw, tmph
|
||||
|
||||
|
||||
# function [bboxA] = rerec(bboxA)
|
||||
def rerec(bboxA):
|
||||
"""Convert bboxA to square."""
|
||||
h = bboxA[:, 3] - bboxA[:, 1]
|
||||
w = bboxA[:, 2] - bboxA[:, 0]
|
||||
l = np.maximum(w, h)
|
||||
bboxA[:, 0] = bboxA[:, 0] + w * 0.5 - l * 0.5
|
||||
bboxA[:, 1] = bboxA[:, 1] + h * 0.5 - l * 0.5
|
||||
bboxA[:, 2:4] = bboxA[:, 0:2] + np.transpose(np.tile(l, (2, 1)))
|
||||
return bboxA
|
||||
|
||||
|
||||
def imresample(img, sz):
|
||||
from keras.preprocessing import image
|
||||
# im_data = resize(img, (sz[0], sz[1]))
|
||||
im_data = image.array_to_img(img).resize((sz[1], sz[0]))
|
||||
im_data = image.img_to_array(im_data)
|
||||
return im_data
|
||||
|
||||
# def imresample(img, sz):
|
||||
# import cv2
|
||||
# im_data = cv2.resize(img, (sz[1], sz[0]), interpolation=cv2.INTER_AREA) # @UndefinedVariable
|
||||
# return im_data
|
||||
|
||||
|
||||
def to_rgb(img):
|
||||
w, h = img.shape
|
||||
ret = np.empty((w, h, 3), dtype=np.uint8)
|
||||
ret[:, :, 0] = ret[:, :, 1] = ret[:, :, 2] = img
|
||||
return ret
|
@ -1,12 +1,10 @@
|
||||
#!/usr/bin/env python
|
||||
# -*- coding: utf-8 -*-
|
||||
# @Date : 2020-05-17
|
||||
# @Author : Shawn Shan (shansixiong@cs.uchicago.edu)
|
||||
# @Link : https://www.shawnshan.com/
|
||||
# @Date : 2020-10-21
|
||||
# @Author : Emily Wenger (ewenger@uchicago.edu)
|
||||
|
||||
import datetime
|
||||
import time
|
||||
from decimal import Decimal
|
||||
|
||||
import numpy as np
|
||||
import tensorflow as tf
|
||||
@ -31,30 +29,27 @@ class FawkesMaskGeneration:
|
||||
KEEP_FINAL = False
|
||||
# max_val of image
|
||||
MAX_VAL = 255
|
||||
# The following variables are used by DSSIM, should keep as default
|
||||
# filter size in SSIM
|
||||
FILTER_SIZE = 11
|
||||
# filter sigma in SSIM
|
||||
FILTER_SIGMA = 1.5
|
||||
# weights used in MS-SSIM
|
||||
SCALE_WEIGHTS = None
|
||||
MAXIMIZE = False
|
||||
IMAGE_SHAPE = (224, 224, 3)
|
||||
IMAGE_SHAPE = (112, 112, 3)
|
||||
RATIO = 1.0
|
||||
LIMIT_DIST = False
|
||||
LOSS_TYPE = 'features' # use features (original Fawkes) or gradients (Witches Brew) to run Fawkes?
|
||||
|
||||
def __init__(self, sess, bottleneck_model_ls, mimic_img=MIMIC_IMG,
|
||||
def __init__(self, bottleneck_model_ls, mimic_img=MIMIC_IMG,
|
||||
batch_size=1, learning_rate=LEARNING_RATE,
|
||||
max_iterations=MAX_ITERATIONS, initial_const=INITIAL_CONST,
|
||||
intensity_range=INTENSITY_RANGE, l_threshold=L_THRESHOLD,
|
||||
max_val=MAX_VAL, keep_final=KEEP_FINAL, maximize=MAXIMIZE, image_shape=IMAGE_SHAPE,
|
||||
verbose=0, ratio=RATIO, limit_dist=LIMIT_DIST):
|
||||
max_val=MAX_VAL, keep_final=KEEP_FINAL, maximize=MAXIMIZE, image_shape=IMAGE_SHAPE, verbose=1,
|
||||
ratio=RATIO, limit_dist=LIMIT_DIST, loss_method=LOSS_TYPE, tanh_process=True,
|
||||
save_last_on_failed=True):
|
||||
|
||||
assert intensity_range in {'raw', 'imagenet', 'inception', 'mnist'}
|
||||
|
||||
# constant used for tanh transformation to avoid corner cases
|
||||
|
||||
self.it = 0
|
||||
self.tanh_constant = 2 - 1e-6
|
||||
self.sess = sess
|
||||
self.save_last_on_failed = save_last_on_failed
|
||||
self.MIMIC_IMG = mimic_img
|
||||
self.LEARNING_RATE = learning_rate
|
||||
self.MAX_ITERATIONS = max_iterations
|
||||
@ -70,350 +65,234 @@ class FawkesMaskGeneration:
|
||||
self.ratio = ratio
|
||||
self.limit_dist = limit_dist
|
||||
self.single_shape = list(image_shape)
|
||||
self.bottleneck_models = bottleneck_model_ls
|
||||
self.loss_method = loss_method
|
||||
self.tanh_process = tanh_process
|
||||
|
||||
self.input_shape = tuple([self.batch_size] + self.single_shape)
|
||||
|
||||
self.bottleneck_shape = tuple([self.batch_size] + self.single_shape)
|
||||
|
||||
# self.bottleneck_shape = tuple([self.batch_size, bottleneck_model_ls[0].output_shape[-1]])
|
||||
|
||||
# the variable we're going to optimize over
|
||||
self.modifier = tf.Variable(np.zeros(self.input_shape, dtype=np.float32))
|
||||
|
||||
# target image in tanh space
|
||||
if self.MIMIC_IMG:
|
||||
self.timg_tanh = tf.Variable(np.zeros(self.input_shape), dtype=np.float32)
|
||||
else:
|
||||
self.bottleneck_t_raw = tf.Variable(np.zeros(self.bottleneck_shape), dtype=np.float32)
|
||||
# source image in tanh space
|
||||
self.simg_tanh = tf.Variable(np.zeros(self.input_shape), dtype=np.float32)
|
||||
|
||||
self.const = tf.Variable(np.ones(batch_size), dtype=np.float32)
|
||||
self.mask = tf.Variable(np.ones((batch_size), dtype=np.bool))
|
||||
self.weights = tf.Variable(np.ones(self.bottleneck_shape,
|
||||
dtype=np.float32))
|
||||
|
||||
# and here's what we use to assign them
|
||||
self.assign_modifier = tf.placeholder(tf.float32, self.input_shape)
|
||||
if self.MIMIC_IMG:
|
||||
self.assign_timg_tanh = tf.placeholder(
|
||||
tf.float32, self.input_shape)
|
||||
else:
|
||||
self.assign_bottleneck_t_raw = tf.placeholder(
|
||||
tf.float32, self.bottleneck_shape)
|
||||
self.assign_simg_tanh = tf.placeholder(tf.float32, self.input_shape)
|
||||
self.assign_const = tf.placeholder(tf.float32, (batch_size))
|
||||
self.assign_mask = tf.placeholder(tf.bool, (batch_size))
|
||||
self.assign_weights = tf.placeholder(tf.float32, self.bottleneck_shape)
|
||||
|
||||
# the resulting image, tanh'd to keep bounded from -0.5 to 0.5
|
||||
# adversarial image in raw space
|
||||
self.aimg_raw = (tf.tanh(self.modifier + self.simg_tanh) /
|
||||
self.tanh_constant +
|
||||
0.5) * 255.0
|
||||
# source image in raw space
|
||||
self.simg_raw = (tf.tanh(self.simg_tanh) /
|
||||
self.tanh_constant +
|
||||
0.5) * 255.0
|
||||
if self.MIMIC_IMG:
|
||||
# target image in raw space
|
||||
self.timg_raw = (tf.tanh(self.timg_tanh) /
|
||||
self.tanh_constant +
|
||||
0.5) * 255.0
|
||||
|
||||
# convert source and adversarial image into input space
|
||||
if self.intensity_range == 'imagenet':
|
||||
mean = tf.constant(np.repeat([[[[103.939, 116.779, 123.68]]]], self.batch_size, axis=0), dtype=tf.float32,
|
||||
name='img_mean')
|
||||
self.aimg_input = (self.aimg_raw[..., ::-1] - mean)
|
||||
self.simg_input = (self.simg_raw[..., ::-1] - mean)
|
||||
if self.MIMIC_IMG:
|
||||
self.timg_input = (self.timg_raw[..., ::-1] - mean)
|
||||
|
||||
elif self.intensity_range == 'raw':
|
||||
self.aimg_input = self.aimg_raw
|
||||
self.simg_input = self.simg_raw
|
||||
if self.MIMIC_IMG:
|
||||
self.timg_input = self.timg_raw
|
||||
|
||||
def batch_gen_DSSIM(aimg_raw_split, simg_raw_split):
|
||||
msssim_split = tf.image.ssim(aimg_raw_split, simg_raw_split, max_val=255.0)
|
||||
dist = (1.0 - tf.stack(msssim_split)) / 2.0
|
||||
# dist = tf.square(aimg_raw_split - simg_raw_split)
|
||||
return dist
|
||||
|
||||
# raw value of DSSIM distance
|
||||
self.dist_raw = batch_gen_DSSIM(self.aimg_raw, self.simg_raw)
|
||||
# distance value after applying threshold
|
||||
self.dist = tf.maximum(self.dist_raw - self.l_threshold, 0.0)
|
||||
# self.dist = self.dist_raw
|
||||
self.dist_raw_sum = tf.reduce_sum(
|
||||
tf.where(self.mask,
|
||||
self.dist_raw,
|
||||
tf.zeros_like(self.dist_raw)))
|
||||
self.dist_sum = tf.reduce_sum(tf.where(self.mask, self.dist, tf.zeros_like(self.dist)))
|
||||
|
||||
def resize_tensor(input_tensor, model_input_shape):
|
||||
if input_tensor.shape[1:] == model_input_shape or model_input_shape[1] is None:
|
||||
return input_tensor
|
||||
resized_tensor = tf.image.resize(input_tensor, model_input_shape[:2])
|
||||
return resized_tensor
|
||||
|
||||
def calculate_direction(bottleneck_model, cur_timg_input, cur_simg_input):
|
||||
target_features = bottleneck_model(cur_timg_input)
|
||||
# return target_features
|
||||
target_center = tf.reduce_mean(target_features, axis=0)
|
||||
original = bottleneck_model(cur_simg_input)
|
||||
original_center = tf.reduce_mean(original, axis=0)
|
||||
direction = target_center - original_center
|
||||
final_target = original + 2.0 * direction
|
||||
return final_target
|
||||
|
||||
self.bottlesim = 0.0
|
||||
self.bottlesim_sum = 0.0
|
||||
self.bottlesim_push = 0.0
|
||||
for bottleneck_model in bottleneck_model_ls:
|
||||
model_input_shape = (224, 224, 3)
|
||||
|
||||
cur_aimg_input = resize_tensor(self.aimg_input, model_input_shape)
|
||||
|
||||
self.bottleneck_a = bottleneck_model(cur_aimg_input)
|
||||
if self.MIMIC_IMG:
|
||||
cur_timg_input = self.timg_input
|
||||
cur_simg_input = self.simg_input
|
||||
self.bottleneck_t = calculate_direction(bottleneck_model, cur_timg_input, cur_simg_input)
|
||||
else:
|
||||
self.bottleneck_t = self.bottleneck_t_raw
|
||||
|
||||
bottleneck_diff = self.bottleneck_t - self.bottleneck_a
|
||||
|
||||
scale_factor = tf.sqrt(tf.reduce_sum(tf.square(self.bottleneck_t), axis=1))
|
||||
|
||||
cur_bottlesim = tf.sqrt(tf.reduce_sum(tf.square(bottleneck_diff), axis=1))
|
||||
cur_bottlesim = cur_bottlesim / scale_factor
|
||||
cur_bottlesim_sum = tf.reduce_sum(cur_bottlesim)
|
||||
|
||||
self.bottlesim += cur_bottlesim
|
||||
|
||||
self.bottlesim_sum += cur_bottlesim_sum
|
||||
|
||||
# sum up the losses
|
||||
if self.maximize:
|
||||
self.loss = self.const * tf.square(self.dist) - self.bottlesim
|
||||
else:
|
||||
self.loss = self.const * tf.square(self.dist) + self.bottlesim
|
||||
|
||||
self.loss_sum = tf.reduce_sum(tf.where(self.mask,
|
||||
self.loss,
|
||||
tf.zeros_like(self.loss)))
|
||||
|
||||
start_vars = set(x.name for x in tf.global_variables())
|
||||
self.learning_rate_holder = tf.placeholder(tf.float32, shape=[])
|
||||
|
||||
optimizer = tf.train.AdadeltaOptimizer(self.learning_rate_holder)
|
||||
# optimizer = tf.train.AdamOptimizer(self.learning_rate_holder)
|
||||
|
||||
self.train = optimizer.minimize(self.loss_sum, var_list=[self.modifier])
|
||||
end_vars = tf.global_variables()
|
||||
new_vars = [x for x in end_vars if x.name not in start_vars]
|
||||
|
||||
# these are the variables to initialize when we run
|
||||
self.setup = []
|
||||
self.setup.append(self.modifier.assign(self.assign_modifier))
|
||||
if self.MIMIC_IMG:
|
||||
self.setup.append(self.timg_tanh.assign(self.assign_timg_tanh))
|
||||
else:
|
||||
self.setup.append(self.bottleneck_t_raw.assign(
|
||||
self.assign_bottleneck_t_raw))
|
||||
self.setup.append(self.simg_tanh.assign(self.assign_simg_tanh))
|
||||
self.setup.append(self.const.assign(self.assign_const))
|
||||
self.setup.append(self.mask.assign(self.assign_mask))
|
||||
self.setup.append(self.weights.assign(self.assign_weights))
|
||||
|
||||
self.init = tf.variables_initializer(var_list=[self.modifier] + new_vars)
|
||||
@staticmethod
|
||||
def resize_tensor(input_tensor, model_input_shape):
|
||||
if input_tensor.shape[1:] == model_input_shape or model_input_shape[1] is None:
|
||||
return input_tensor
|
||||
resized_tensor = tf.image.resize(input_tensor, model_input_shape[:2])
|
||||
return resized_tensor
|
||||
|
||||
def preprocess_arctanh(self, imgs):
|
||||
|
||||
""" Do tan preprocess """
|
||||
imgs = reverse_preprocess(imgs, self.intensity_range)
|
||||
imgs /= 255.0
|
||||
imgs -= 0.5
|
||||
imgs *= self.tanh_constant
|
||||
imgs = imgs / 255.0
|
||||
imgs = imgs - 0.5
|
||||
imgs = imgs * self.tanh_constant
|
||||
tanh_imgs = np.arctanh(imgs)
|
||||
|
||||
return tanh_imgs
|
||||
|
||||
def clipping(self, imgs):
|
||||
def reverse_arctanh(self, imgs):
|
||||
raw_img = (tf.tanh(imgs) / self.tanh_constant + 0.5) * 255
|
||||
return raw_img
|
||||
|
||||
def input_space_process(self, img):
|
||||
if self.intensity_range == 'imagenet':
|
||||
mean = np.repeat([[[[103.939, 116.779, 123.68]]]], len(img), axis=0)
|
||||
raw_img = (img[..., ::-1] - mean)
|
||||
else:
|
||||
raw_img = img
|
||||
return raw_img
|
||||
|
||||
def clipping(self, imgs):
|
||||
imgs = reverse_preprocess(imgs, self.intensity_range)
|
||||
imgs = np.clip(imgs, 0, self.max_val)
|
||||
imgs = preprocess(imgs, self.intensity_range)
|
||||
|
||||
return imgs
|
||||
|
||||
def attack(self, source_imgs, target_imgs, weights=None):
|
||||
def calc_dissim(self, source_raw, source_mod_raw):
|
||||
msssim_split = tf.image.ssim(source_raw, source_mod_raw, max_val=255.0)
|
||||
dist_raw = (1.0 - tf.stack(msssim_split)) / 2.0
|
||||
dist = tf.maximum(dist_raw - self.l_threshold, 0.0)
|
||||
dist_raw_avg = tf.reduce_mean(dist_raw)
|
||||
dist_sum = tf.reduce_sum(dist)
|
||||
|
||||
if weights is None:
|
||||
weights = np.ones([source_imgs.shape[0]] +
|
||||
list(self.bottleneck_shape[1:]))
|
||||
return dist, dist_raw, dist_sum, dist_raw_avg
|
||||
|
||||
assert weights.shape[1:] == self.bottleneck_shape[1:]
|
||||
assert source_imgs.shape[1:] == self.input_shape[1:]
|
||||
assert source_imgs.shape[0] == weights.shape[0]
|
||||
if self.MIMIC_IMG:
|
||||
assert target_imgs.shape[1:] == self.input_shape[1:]
|
||||
assert source_imgs.shape[0] == target_imgs.shape[0]
|
||||
def calc_bottlesim(self, tape, source_raw, target_raw, original_raw):
|
||||
""" original Fawkes loss function. """
|
||||
bottlesim = 0.0
|
||||
bottlesim_sum = 0.0
|
||||
# make sure everything is the right size.
|
||||
model_input_shape = self.single_shape
|
||||
cur_aimg_input = self.resize_tensor(source_raw, model_input_shape)
|
||||
if target_raw is not None:
|
||||
cur_timg_input = self.resize_tensor(target_raw, model_input_shape)
|
||||
for bottleneck_model in self.bottleneck_models:
|
||||
if tape is not None:
|
||||
try:
|
||||
tape.watch(bottleneck_model.model.variables)
|
||||
except AttributeError:
|
||||
tape.watch(bottleneck_model.variables)
|
||||
# get the respective feature space reprs.
|
||||
bottleneck_a = bottleneck_model(cur_aimg_input)
|
||||
if self.maximize:
|
||||
bottleneck_s = bottleneck_model(original_raw)
|
||||
bottleneck_diff = bottleneck_a - bottleneck_s
|
||||
scale_factor = tf.sqrt(tf.reduce_sum(tf.square(bottleneck_s), axis=1))
|
||||
else:
|
||||
bottleneck_t = bottleneck_model(cur_timg_input)
|
||||
bottleneck_diff = bottleneck_t - bottleneck_a
|
||||
scale_factor = tf.sqrt(tf.reduce_sum(tf.square(bottleneck_t), axis=1))
|
||||
cur_bottlesim = tf.reduce_sum(tf.square(bottleneck_diff), axis=1)
|
||||
cur_bottlesim = cur_bottlesim / scale_factor
|
||||
bottlesim += cur_bottlesim
|
||||
bottlesim_sum += tf.reduce_sum(cur_bottlesim)
|
||||
return bottlesim, bottlesim_sum
|
||||
|
||||
def compute_feature_loss(self, tape, aimg_raw, simg_raw, aimg_input, timg_input, simg_input):
|
||||
""" Compute input space + feature space loss.
|
||||
"""
|
||||
input_space_loss, dist_raw, input_space_loss_sum, input_space_loss_raw_avg = self.calc_dissim(aimg_raw,
|
||||
simg_raw)
|
||||
feature_space_loss, feature_space_loss_sum = self.calc_bottlesim(tape, aimg_input, timg_input, simg_input)
|
||||
|
||||
if self.maximize:
|
||||
loss = self.const * tf.square(input_space_loss) - feature_space_loss * self.const_diff
|
||||
else:
|
||||
assert target_imgs.shape[1:] == self.bottleneck_shape[1:]
|
||||
assert source_imgs.shape[0] == target_imgs.shape[0]
|
||||
if self.it < self.MAX_ITERATIONS:
|
||||
loss = self.const * tf.square(input_space_loss) + 1000 * feature_space_loss
|
||||
|
||||
loss_sum = tf.reduce_sum(loss)
|
||||
return loss_sum, feature_space_loss, input_space_loss_raw_avg, dist_raw
|
||||
|
||||
def compute(self, source_imgs, target_imgs=None):
|
||||
""" Main function that runs cloak generation. """
|
||||
start_time = time.time()
|
||||
|
||||
adv_imgs = []
|
||||
print('%d batches in total'
|
||||
% int(np.ceil(len(source_imgs) / self.batch_size)))
|
||||
|
||||
for idx in range(0, len(source_imgs), self.batch_size):
|
||||
print('processing image %d at %s' % (idx+1, datetime.datetime.now()))
|
||||
adv_img = self.attack_batch(source_imgs[idx:idx + self.batch_size],
|
||||
target_imgs[idx:idx + self.batch_size],
|
||||
weights[idx:idx + self.batch_size])
|
||||
print('processing image %d at %s' % (idx + 1, datetime.datetime.now()))
|
||||
adv_img = self.compute_batch(source_imgs[idx:idx + self.batch_size],
|
||||
target_imgs[idx:idx + self.batch_size] if target_imgs is not None else None)
|
||||
adv_imgs.extend(adv_img)
|
||||
|
||||
elapsed_time = time.time() - start_time
|
||||
print('protection cost %f s' % (elapsed_time))
|
||||
|
||||
print('protection cost %f s' % elapsed_time)
|
||||
return np.array(adv_imgs)
|
||||
|
||||
def attack_batch(self, source_imgs, target_imgs, weights):
|
||||
|
||||
LR = self.learning_rate
|
||||
def compute_batch(self, source_imgs, target_imgs=None, retry=True):
|
||||
""" TF2 method to generate the cloak. """
|
||||
# preprocess images.
|
||||
global progressbar
|
||||
nb_imgs = source_imgs.shape[0]
|
||||
mask = [True] * nb_imgs + [False] * (self.batch_size - nb_imgs)
|
||||
mask = np.array(mask, dtype=np.bool)
|
||||
|
||||
source_imgs = np.array(source_imgs)
|
||||
target_imgs = np.array(target_imgs)
|
||||
# make sure source/target images are an array
|
||||
source_imgs = np.array(source_imgs, dtype=np.float32)
|
||||
if target_imgs is not None:
|
||||
target_imgs = np.array(target_imgs, dtype=np.float32)
|
||||
|
||||
# metrics to test
|
||||
best_bottlesim = [0] * nb_imgs if self.maximize else [np.inf] * nb_imgs
|
||||
best_adv = np.zeros(source_imgs.shape)
|
||||
|
||||
# convert to tanh-space
|
||||
simg_tanh = self.preprocess_arctanh(source_imgs)
|
||||
if self.MIMIC_IMG:
|
||||
if target_imgs is not None:
|
||||
timg_tanh = self.preprocess_arctanh(target_imgs)
|
||||
else:
|
||||
timg_tanh = target_imgs
|
||||
self.modifier = tf.Variable(np.ones(tuple([len(source_imgs)] + self.single_shape), dtype=np.float32) * 1e-4)
|
||||
|
||||
CONST = np.ones(self.batch_size) * self.initial_const
|
||||
# make the optimizer
|
||||
optimizer = tf.keras.optimizers.Adadelta(float(self.learning_rate))
|
||||
const_numpy = np.ones(len(source_imgs)) * self.initial_const
|
||||
self.const = tf.Variable(const_numpy, dtype=np.float32)
|
||||
|
||||
self.sess.run(self.init)
|
||||
simg_tanh_batch = np.zeros(self.input_shape)
|
||||
if self.MIMIC_IMG:
|
||||
timg_tanh_batch = np.zeros(self.input_shape)
|
||||
else:
|
||||
timg_tanh_batch = np.zeros(self.bottleneck_shape)
|
||||
|
||||
weights_batch = np.zeros(self.bottleneck_shape)
|
||||
simg_tanh_batch[:nb_imgs] = simg_tanh[:nb_imgs]
|
||||
timg_tanh_batch[:nb_imgs] = timg_tanh[:nb_imgs]
|
||||
weights_batch[:nb_imgs] = weights[:nb_imgs]
|
||||
modifier_batch = np.ones(self.input_shape) * 1e-6
|
||||
|
||||
# set the variables so that we don't have to send them over again
|
||||
if self.MIMIC_IMG:
|
||||
self.sess.run(self.setup,
|
||||
{self.assign_timg_tanh: timg_tanh_batch,
|
||||
self.assign_simg_tanh: simg_tanh_batch,
|
||||
self.assign_const: CONST,
|
||||
self.assign_mask: mask,
|
||||
self.assign_weights: weights_batch,
|
||||
self.assign_modifier: modifier_batch})
|
||||
|
||||
best_bottlesim = [0] * nb_imgs if self.maximize else [np.inf] * nb_imgs
|
||||
best_adv = np.zeros_like(source_imgs)
|
||||
|
||||
if self.verbose == 1:
|
||||
loss_sum = float(self.sess.run(self.loss_sum))
|
||||
dist_sum = float(self.sess.run(self.dist_sum))
|
||||
thresh_over = (dist_sum / self.batch_size / self.l_threshold * 100)
|
||||
dist_raw_sum = float(self.sess.run(self.dist_raw_sum))
|
||||
bottlesim_sum = self.sess.run(self.bottlesim_sum)
|
||||
print('START: Total loss: %.4E; perturb: %.6f (%.2f%% over, raw: %.6f); sim: %f'
|
||||
% (Decimal(loss_sum),
|
||||
dist_sum,
|
||||
thresh_over,
|
||||
dist_raw_sum,
|
||||
bottlesim_sum / nb_imgs))
|
||||
|
||||
finished_idx = set()
|
||||
total_distance = [0] * nb_imgs
|
||||
|
||||
if self.limit_dist:
|
||||
dist_raw_list, bottlesim_list, aimg_input_list = self.sess.run(
|
||||
[self.dist_raw,
|
||||
self.bottlesim,
|
||||
self.aimg_input])
|
||||
for e, (dist_raw, bottlesim, aimg_input) in enumerate(
|
||||
zip(dist_raw_list, bottlesim_list, aimg_input_list)):
|
||||
if e >= nb_imgs:
|
||||
break
|
||||
total_distance[e] = bottlesim
|
||||
const_diff_numpy = np.ones(len(source_imgs)) * 1.0
|
||||
self.const_diff = tf.Variable(const_diff_numpy, dtype=np.float32)
|
||||
|
||||
# get the modifier
|
||||
if self.verbose == 0:
|
||||
progressbar = Progbar(
|
||||
self.MAX_ITERATIONS, width=30, verbose=1
|
||||
)
|
||||
# watch relevant variables.
|
||||
simg_tanh = tf.Variable(simg_tanh, dtype=np.float32)
|
||||
simg_raw = tf.Variable(source_imgs, dtype=np.float32)
|
||||
if target_imgs is not None:
|
||||
timg_raw = tf.Variable(timg_tanh, dtype=np.float32)
|
||||
# run the attack
|
||||
outside_list = np.ones(len(source_imgs))
|
||||
self.it = 0
|
||||
|
||||
for iteration in range(self.MAX_ITERATIONS):
|
||||
while self.it < self.MAX_ITERATIONS:
|
||||
|
||||
self.sess.run([self.train], feed_dict={self.learning_rate_holder: LR})
|
||||
self.it += 1
|
||||
with tf.GradientTape(persistent=True) as tape:
|
||||
tape.watch(self.modifier)
|
||||
tape.watch(simg_tanh)
|
||||
|
||||
dist_raw_list, bottlesim_list, aimg_input_list = self.sess.run(
|
||||
[self.dist_raw,
|
||||
self.bottlesim,
|
||||
self.aimg_input])
|
||||
# Convert from tanh for DISSIM
|
||||
aimg_raw = self.reverse_arctanh(simg_tanh + self.modifier)
|
||||
|
||||
all_clear = True
|
||||
for e, (dist_raw, bottlesim, aimg_input) in enumerate(
|
||||
zip(dist_raw_list, bottlesim_list, aimg_input_list)):
|
||||
actual_modifier = aimg_raw - simg_raw
|
||||
actual_modifier = tf.clip_by_value(actual_modifier, -15.0, 15.0)
|
||||
aimg_raw = simg_raw + actual_modifier
|
||||
|
||||
if e in finished_idx:
|
||||
continue
|
||||
simg_raw = self.reverse_arctanh(simg_tanh)
|
||||
|
||||
# Convert further preprocess for bottleneck
|
||||
aimg_input = self.input_space_process(aimg_raw)
|
||||
if target_imgs is not None:
|
||||
timg_input = self.input_space_process(timg_raw)
|
||||
else:
|
||||
timg_input = None
|
||||
simg_input = self.input_space_process(simg_raw)
|
||||
|
||||
# get the feature space loss.
|
||||
loss, internal_dist, input_dist_avg, dist_raw = self.compute_feature_loss(
|
||||
tape, aimg_raw, simg_raw, aimg_input, timg_input, simg_input)
|
||||
|
||||
# compute gradients
|
||||
grad = tape.gradient(loss, [self.modifier])
|
||||
optimizer.apply_gradients(zip(grad, [self.modifier]))
|
||||
|
||||
if self.it == 1:
|
||||
self.modifier = tf.Variable(self.modifier - tf.sign(grad[0]) * 0.01, dtype=tf.float32)
|
||||
|
||||
for e, (input_dist, feature_d, mod_img) in enumerate(zip(dist_raw, internal_dist, aimg_input)):
|
||||
if e >= nb_imgs:
|
||||
break
|
||||
if (bottlesim < best_bottlesim[e] and bottlesim > total_distance[e] * 0.1 and (
|
||||
not self.maximize)) or (
|
||||
bottlesim > best_bottlesim[e] and self.maximize):
|
||||
best_bottlesim[e] = bottlesim
|
||||
best_adv[e] = aimg_input
|
||||
input_dist = input_dist.numpy()
|
||||
feature_d = feature_d.numpy()
|
||||
|
||||
all_clear = False
|
||||
if input_dist <= self.l_threshold * 0.9 and const_diff_numpy[e] <= 129:
|
||||
const_diff_numpy[e] *= 2
|
||||
if outside_list[e] == -1:
|
||||
const_diff_numpy[e] = 1
|
||||
outside_list[e] = 1
|
||||
elif input_dist >= self.l_threshold * 1.1 and const_diff_numpy[e] >= 1 / 129:
|
||||
const_diff_numpy[e] /= 2
|
||||
|
||||
if all_clear:
|
||||
break
|
||||
if outside_list[e] == 1:
|
||||
const_diff_numpy[e] = 1
|
||||
outside_list[e] = -1
|
||||
else:
|
||||
const_diff_numpy[e] = 1.0
|
||||
outside_list[e] = 0
|
||||
|
||||
if iteration != 0 and iteration % (self.MAX_ITERATIONS // 3) == 0:
|
||||
LR = LR * 0.8
|
||||
if self.verbose:
|
||||
print("Learning rate: ", LR)
|
||||
if input_dist <= self.l_threshold * 1.1 and (
|
||||
(feature_d < best_bottlesim[e] and (not self.maximize)) or (
|
||||
feature_d > best_bottlesim[e] and self.maximize)):
|
||||
best_bottlesim[e] = feature_d
|
||||
best_adv[e] = mod_img
|
||||
|
||||
self.const_diff = tf.Variable(const_diff_numpy, dtype=np.float32)
|
||||
|
||||
if self.verbose == 1:
|
||||
print("ITER {:0.2f} Total Loss: {:.2f} {:0.4f} raw; diff: {:.4f}".format(self.it, loss, input_dist_avg,
|
||||
np.mean(internal_dist)))
|
||||
|
||||
if iteration % (self.MAX_ITERATIONS // 5) == 0:
|
||||
if self.verbose == 1:
|
||||
dist_raw_sum = float(self.sess.run(self.dist_raw_sum))
|
||||
bottlesim_sum = self.sess.run(self.bottlesim_sum)
|
||||
print('ITER %4d perturb: %.5f; sim: %f'
|
||||
% (iteration, dist_raw_sum / nb_imgs, bottlesim_sum / nb_imgs))
|
||||
if self.verbose == 0:
|
||||
progressbar.update(iteration)
|
||||
|
||||
progressbar.update(self.it)
|
||||
if self.verbose == 1:
|
||||
loss_sum = float(self.sess.run(self.loss_sum))
|
||||
dist_sum = float(self.sess.run(self.dist_sum))
|
||||
dist_raw_sum = float(self.sess.run(self.dist_raw_sum))
|
||||
bottlesim_sum = float(self.sess.run(self.bottlesim_sum))
|
||||
print('END: Total loss: %.4E; perturb: %.6f (raw: %.6f); sim: %f'
|
||||
% (Decimal(loss_sum),
|
||||
dist_sum,
|
||||
dist_raw_sum,
|
||||
bottlesim_sum / nb_imgs))
|
||||
print("Final diff: {:.4f}".format(np.mean(best_bottlesim)))
|
||||
print("\n")
|
||||
|
||||
if self.save_last_on_failed:
|
||||
for e, diff in enumerate(best_bottlesim):
|
||||
if diff < 0.3 and dist_raw[e] < 0.015 and internal_dist[e] > diff:
|
||||
best_adv[e] = aimg_input[e]
|
||||
|
||||
best_adv = self.clipping(best_adv[:nb_imgs])
|
||||
return best_adv
|
||||
|
@ -10,87 +10,83 @@ import logging
|
||||
import os
|
||||
import sys
|
||||
|
||||
logging.getLogger('tensorflow').setLevel(logging.ERROR)
|
||||
os.environ["KMP_AFFINITY"] = "noverbose"
|
||||
os.environ['TF_CPP_MIN_LOG_LEVEL'] = '3'
|
||||
import tensorflow as tf
|
||||
|
||||
logging.getLogger('tensorflow').disabled = True
|
||||
tf.get_logger().setLevel('ERROR')
|
||||
tf.autograph.set_verbosity(3)
|
||||
|
||||
import numpy as np
|
||||
from fawkes.differentiator import FawkesMaskGeneration
|
||||
from fawkes.utils import load_extractor, init_gpu, select_target_label, dump_image, reverse_process_cloaked, \
|
||||
Faces, filter_image_paths
|
||||
from fawkes.utils import init_gpu, dump_image, reverse_process_cloaked, \
|
||||
Faces, filter_image_paths, load_extractor
|
||||
|
||||
from fawkes.align_face import aligner
|
||||
from fawkes.utils import get_file
|
||||
|
||||
|
||||
def generate_cloak_images(protector, image_X, target_emb=None):
|
||||
cloaked_image_X = protector.attack(image_X, target_emb)
|
||||
cloaked_image_X = protector.compute(image_X, target_emb)
|
||||
return cloaked_image_X
|
||||
|
||||
|
||||
IMG_SIZE = 112
|
||||
PREPROCESS = 'raw'
|
||||
|
||||
|
||||
class Fawkes(object):
|
||||
def __init__(self, feature_extractor, gpu, batch_size):
|
||||
def __init__(self, feature_extractor, gpu, batch_size, mode="low"):
|
||||
|
||||
self.feature_extractor = feature_extractor
|
||||
self.gpu = gpu
|
||||
self.batch_size = batch_size
|
||||
global sess
|
||||
sess = init_gpu(gpu)
|
||||
global graph
|
||||
graph = tf.get_default_graph()
|
||||
self.mode = mode
|
||||
th, max_step, lr, extractors = self.mode2param(self.mode)
|
||||
self.th = th
|
||||
self.lr = lr
|
||||
self.max_step = max_step
|
||||
|
||||
model_dir = os.path.join(os.path.expanduser('~'), '.fawkes')
|
||||
if not os.path.exists(os.path.join(model_dir, "mtcnn.p.gz")):
|
||||
os.makedirs(model_dir, exist_ok=True)
|
||||
get_file("mtcnn.p.gz", "http://mirror.cs.uchicago.edu/fawkes/files/mtcnn.p.gz", cache_dir=model_dir,
|
||||
cache_subdir='')
|
||||
init_gpu(gpu)
|
||||
|
||||
self.fs_names = [feature_extractor]
|
||||
if isinstance(feature_extractor, list):
|
||||
self.fs_names = feature_extractor
|
||||
# model_dir = os.path.join(os.path.expanduser('~'), '.fawkes')
|
||||
# if not os.path.exists(os.path.join(model_dir)):
|
||||
# os.makedirs(model_dir, exist_ok=True)
|
||||
|
||||
self.aligner = aligner(sess)
|
||||
self.feature_extractors_ls = [load_extractor(name) for name in self.fs_names]
|
||||
self.aligner = aligner()
|
||||
|
||||
self.protector = None
|
||||
self.protector_param = None
|
||||
self.feature_extractors_ls = [load_extractor(name) for name in extractors]
|
||||
|
||||
def mode2param(self, mode):
|
||||
if mode == 'min':
|
||||
th = 0.002
|
||||
max_step = 20
|
||||
lr = 40
|
||||
elif mode == 'low':
|
||||
th = 0.003
|
||||
max_step = 50
|
||||
lr = 35
|
||||
elif mode == 'mid':
|
||||
if mode == 'low':
|
||||
th = 0.005
|
||||
max_step = 200
|
||||
lr = 20
|
||||
elif mode == 'high':
|
||||
th = 0.008
|
||||
max_step = 500
|
||||
lr = 10
|
||||
elif mode == 'ultra':
|
||||
if not tf.test.is_gpu_available():
|
||||
print("Please enable GPU for ultra setting...")
|
||||
sys.exit(1)
|
||||
max_step = 25
|
||||
lr = 25
|
||||
extractors = ["extractor_2"]
|
||||
|
||||
elif mode == 'mid':
|
||||
th = 0.01
|
||||
max_step = 1000
|
||||
lr = 8
|
||||
else:
|
||||
raise Exception("mode must be one of 'min', 'low', 'mid', 'high', 'ultra', 'custom'")
|
||||
return th, max_step, lr
|
||||
max_step = 50
|
||||
lr = 20
|
||||
extractors = ["extractor_0", "extractor_2"]
|
||||
|
||||
def run_protection(self, image_paths, mode='min', th=0.04, sd=1e9, lr=10, max_step=500, batch_size=1, format='png',
|
||||
separate_target=True, debug=False, no_align=False):
|
||||
if mode == 'custom':
|
||||
pass
|
||||
else:
|
||||
th, max_step, lr = self.mode2param(mode)
|
||||
elif mode == 'high':
|
||||
th = 0.015
|
||||
max_step = 100
|
||||
lr = 15
|
||||
extractors = ["extractor_0", "extractor_2"]
|
||||
|
||||
current_param = "-".join([str(x) for x in [mode, th, sd, lr, max_step, batch_size, format,
|
||||
else:
|
||||
raise Exception("mode must be one of 'min', 'low', 'mid', 'high'")
|
||||
return th, max_step, lr, extractors
|
||||
|
||||
def run_protection(self, image_paths, th=0.04, sd=1e9, lr=10, max_step=500, batch_size=1, format='png',
|
||||
separate_target=True, debug=False, no_align=False, exp="", maximize=True,
|
||||
save_last_on_failed=True):
|
||||
|
||||
current_param = "-".join([str(x) for x in [self.th, sd, self.lr, self.max_step, batch_size, format,
|
||||
separate_target, debug]])
|
||||
|
||||
image_paths, loaded_images = filter_image_paths(image_paths)
|
||||
@ -99,55 +95,49 @@ class Fawkes(object):
|
||||
print("No images in the directory")
|
||||
return 3
|
||||
|
||||
with graph.as_default():
|
||||
faces = Faces(image_paths, loaded_images, self.aligner, verbose=1, no_align=no_align)
|
||||
original_images = faces.cropped_faces
|
||||
faces = Faces(image_paths, loaded_images, self.aligner, verbose=1, no_align=no_align)
|
||||
original_images = faces.cropped_faces
|
||||
|
||||
if len(original_images) == 0:
|
||||
print("No face detected. ")
|
||||
return 2
|
||||
original_images = np.array(original_images)
|
||||
if len(original_images) == 0:
|
||||
print("No face detected. ")
|
||||
return 2
|
||||
original_images = np.array(original_images)
|
||||
|
||||
with sess.as_default():
|
||||
if separate_target:
|
||||
target_embedding = []
|
||||
for org_img in original_images:
|
||||
org_img = org_img.reshape([1] + list(org_img.shape))
|
||||
tar_emb = select_target_label(org_img, self.feature_extractors_ls, self.fs_names)
|
||||
target_embedding.append(tar_emb)
|
||||
target_embedding = np.concatenate(target_embedding)
|
||||
else:
|
||||
target_embedding = select_target_label(original_images, self.feature_extractors_ls, self.fs_names)
|
||||
if current_param != self.protector_param:
|
||||
self.protector_param = current_param
|
||||
if self.protector is not None:
|
||||
del self.protector
|
||||
if batch_size == -1:
|
||||
batch_size = len(original_images)
|
||||
self.protector = FawkesMaskGeneration(self.feature_extractors_ls,
|
||||
batch_size=batch_size,
|
||||
mimic_img=True,
|
||||
intensity_range=PREPROCESS,
|
||||
initial_const=sd,
|
||||
learning_rate=self.lr,
|
||||
max_iterations=self.max_step,
|
||||
l_threshold=self.th,
|
||||
verbose=0,
|
||||
maximize=maximize,
|
||||
keep_final=False,
|
||||
image_shape=(IMG_SIZE, IMG_SIZE, 3),
|
||||
loss_method='features',
|
||||
tanh_process=True,
|
||||
save_last_on_failed=save_last_on_failed,
|
||||
)
|
||||
protected_images = generate_cloak_images(self.protector, original_images)
|
||||
faces.cloaked_cropped_faces = protected_images
|
||||
|
||||
if current_param != self.protector_param:
|
||||
self.protector_param = current_param
|
||||
final_images, images_without_face = faces.merge_faces(
|
||||
reverse_process_cloaked(protected_images, preprocess=PREPROCESS),
|
||||
reverse_process_cloaked(original_images, preprocess=PREPROCESS))
|
||||
|
||||
if self.protector is not None:
|
||||
del self.protector
|
||||
|
||||
self.protector = FawkesMaskGeneration(sess, self.feature_extractors_ls,
|
||||
batch_size=batch_size,
|
||||
mimic_img=True,
|
||||
intensity_range='imagenet',
|
||||
initial_const=sd,
|
||||
learning_rate=lr,
|
||||
max_iterations=max_step,
|
||||
l_threshold=th,
|
||||
verbose=1 if debug else 0,
|
||||
maximize=False,
|
||||
keep_final=False,
|
||||
image_shape=(224, 224, 3))
|
||||
|
||||
protected_images = generate_cloak_images(self.protector, original_images,
|
||||
target_emb=target_embedding)
|
||||
|
||||
faces.cloaked_cropped_faces = protected_images
|
||||
|
||||
final_images = faces.merge_faces(reverse_process_cloaked(protected_images),
|
||||
reverse_process_cloaked(original_images))
|
||||
|
||||
for p_img, path in zip(final_images, image_paths):
|
||||
file_name = "{}_{}_cloaked.{}".format(".".join(path.split(".")[:-1]), mode, format)
|
||||
for i in range(len(final_images)):
|
||||
if i in images_without_face:
|
||||
continue
|
||||
p_img = final_images[i]
|
||||
path = image_paths[i]
|
||||
file_name = "{}_cloaked.{}".format(".".join(path.split(".")[:-1]), format)
|
||||
dump_image(p_img, file_name, format=format)
|
||||
|
||||
print("Done!")
|
||||
@ -167,26 +157,22 @@ def main(*argv):
|
||||
parser = argparse.ArgumentParser()
|
||||
parser.add_argument('--directory', '-d', type=str,
|
||||
help='the directory that contains images to run protection', default='imgs/')
|
||||
|
||||
parser.add_argument('--gpu', '-g', type=str,
|
||||
help='the GPU id when using GPU for optimization', default='0')
|
||||
|
||||
parser.add_argument('--mode', '-m', type=str,
|
||||
help='cloak generation mode, select from min, low, mid, high. The higher the mode is, the more perturbation added and stronger protection',
|
||||
default='min')
|
||||
|
||||
help='cloak generation mode, select from min, low, mid, high. The higher the mode is, '
|
||||
'the more perturbation added and stronger protection',
|
||||
default='low')
|
||||
parser.add_argument('--feature-extractor', type=str,
|
||||
help="name of the feature extractor used for optimization, currently only support high_extract",
|
||||
default="high_extract")
|
||||
|
||||
help="name of the feature extractor used for optimization",
|
||||
default="arcface_extractor_0")
|
||||
parser.add_argument('--th', help='only relevant with mode=custom, DSSIM threshold for perturbation', type=float,
|
||||
default=0.01)
|
||||
parser.add_argument('--max-step', help='only relevant with mode=custom, number of steps for optimization', type=int,
|
||||
default=1000)
|
||||
parser.add_argument('--sd', type=int, help='only relevant with mode=custom, penalty number, read more in the paper',
|
||||
default=1e9)
|
||||
default=1e6)
|
||||
parser.add_argument('--lr', type=float, help='only relevant with mode=custom, learning rate', default=2)
|
||||
|
||||
parser.add_argument('--batch-size', help="number of images to run optimization together", type=int, default=1)
|
||||
parser.add_argument('--separate_target', help="whether select separate targets for each faces in the directory",
|
||||
action='store_true')
|
||||
@ -207,18 +193,12 @@ def main(*argv):
|
||||
image_paths = glob.glob(os.path.join(args.directory, "*"))
|
||||
image_paths = [path for path in image_paths if "_cloaked" not in path.split("/")[-1]]
|
||||
|
||||
protector = Fawkes(args.feature_extractor, args.gpu, args.batch_size)
|
||||
if args.mode == 'all':
|
||||
for mode in ['min', 'low', 'mid', 'high']:
|
||||
protector.run_protection(image_paths, mode=mode, th=args.th, sd=args.sd, lr=args.lr,
|
||||
max_step=args.max_step,
|
||||
batch_size=args.batch_size, format=args.format,
|
||||
separate_target=args.separate_target, debug=args.debug, no_align=args.no_align)
|
||||
else:
|
||||
protector.run_protection(image_paths, mode=args.mode, th=args.th, sd=args.sd, lr=args.lr,
|
||||
max_step=args.max_step,
|
||||
batch_size=args.batch_size, format=args.format,
|
||||
separate_target=args.separate_target, debug=args.debug, no_align=args.no_align)
|
||||
protector = Fawkes(args.feature_extractor, args.gpu, args.batch_size, mode=args.mode)
|
||||
|
||||
protector.run_protection(image_paths, th=args.th, sd=args.sd, lr=args.lr,
|
||||
max_step=args.max_step,
|
||||
batch_size=args.batch_size, format=args.format,
|
||||
separate_target=args.separate_target, debug=args.debug, no_align=args.no_align)
|
||||
|
||||
|
||||
if __name__ == '__main__':
|
||||
|
211
fawkes/utils.py
211
fawkes/utils.py
@ -8,6 +8,7 @@
|
||||
import errno
|
||||
import glob
|
||||
import gzip
|
||||
import hashlib
|
||||
import json
|
||||
import os
|
||||
import pickle
|
||||
@ -18,7 +19,9 @@ import tarfile
|
||||
import zipfile
|
||||
|
||||
import PIL
|
||||
import pkg_resources
|
||||
import six
|
||||
from keras.utils import Progbar
|
||||
from six.moves.urllib.error import HTTPError, URLError
|
||||
|
||||
stderr = sys.stderr
|
||||
@ -70,6 +73,10 @@ def clip_img(X, preprocessing='raw'):
|
||||
return X
|
||||
|
||||
|
||||
IMG_SIZE = 112
|
||||
PREPROCESS = 'raw'
|
||||
|
||||
|
||||
def load_image(path):
|
||||
try:
|
||||
img = Image.open(path)
|
||||
@ -130,7 +137,9 @@ class Faces(object):
|
||||
self.cropped_faces = []
|
||||
self.cropped_faces_shape = []
|
||||
self.cropped_index = []
|
||||
self.start_end_ls = []
|
||||
self.callback_idx = []
|
||||
self.images_without_face = []
|
||||
for i in range(0, len(loaded_images)):
|
||||
cur_img = loaded_images[i]
|
||||
p = image_paths[i]
|
||||
@ -144,13 +153,15 @@ class Faces(object):
|
||||
if not no_align:
|
||||
align_img = align(cur_img, self.aligner, margin=margin)
|
||||
if align_img is None:
|
||||
print("Find 0 face(s)".format(p.split("/")[-1]))
|
||||
print("Find 0 face(s) in {}".format(p.split("/")[-1]))
|
||||
self.images_without_face.append(i)
|
||||
continue
|
||||
|
||||
cur_faces = align_img[0]
|
||||
else:
|
||||
cur_faces = [cur_img]
|
||||
|
||||
cur_faces = [face for face in cur_faces if face.shape[0] != 0 and face.shape[1] != 0]
|
||||
cur_shapes = [f.shape[:-1] for f in cur_faces]
|
||||
|
||||
cur_faces_square = []
|
||||
@ -161,17 +172,20 @@ class Faces(object):
|
||||
|
||||
for img in cur_faces:
|
||||
if eval_local:
|
||||
base = resize(img, (224, 224))
|
||||
base = resize(img, (IMG_SIZE, IMG_SIZE))
|
||||
else:
|
||||
long_size = max([img.shape[1], img.shape[0]])
|
||||
base = np.zeros((long_size, long_size, 3))
|
||||
# import pdb
|
||||
# pdb.set_trace()
|
||||
base = np.ones((long_size, long_size, 3)) * np.mean(img, axis=(0, 1))
|
||||
|
||||
start1, end1 = get_ends(long_size, img.shape[0])
|
||||
start2, end2 = get_ends(long_size, img.shape[1])
|
||||
|
||||
base[start1:end1, start2:end2, :] = img
|
||||
cur_start_end = (start1, end1, start2, end2)
|
||||
self.start_end_ls.append(cur_start_end)
|
||||
|
||||
base[0:img.shape[0], 0:img.shape[1], :] = img
|
||||
cur_faces_square.append(base)
|
||||
|
||||
cur_faces_square = [resize(f, (224, 224)) for f in cur_faces_square]
|
||||
cur_faces_square = [resize(f, (IMG_SIZE, IMG_SIZE)) for f in cur_faces_square]
|
||||
self.cropped_faces.extend(cur_faces_square)
|
||||
|
||||
if not self.no_align:
|
||||
@ -187,7 +201,7 @@ class Faces(object):
|
||||
self.cropped_faces = np.array(self.cropped_faces)
|
||||
|
||||
if preprocessing:
|
||||
self.cropped_faces = preprocess(self.cropped_faces, 'imagenet')
|
||||
self.cropped_faces = preprocess(self.cropped_faces, PREPROCESS)
|
||||
|
||||
self.cloaked_cropped_faces = None
|
||||
self.cloaked_faces = np.copy(self.org_faces)
|
||||
@ -197,7 +211,7 @@ class Faces(object):
|
||||
|
||||
def merge_faces(self, protected_images, original_images):
|
||||
if self.no_align:
|
||||
return np.clip(protected_images, 0.0, 255.0)
|
||||
return np.clip(protected_images, 0.0, 255.0), self.images_without_face
|
||||
|
||||
self.cloaked_faces = np.copy(self.org_faces)
|
||||
|
||||
@ -206,22 +220,29 @@ class Faces(object):
|
||||
cur_original = original_images[i]
|
||||
|
||||
org_shape = self.cropped_faces_shape[i]
|
||||
old_square_shape = max([org_shape[0], org_shape[1]])
|
||||
|
||||
old_square_shape = max([org_shape[0], org_shape[1]])
|
||||
cur_protected = resize(cur_protected, (old_square_shape, old_square_shape))
|
||||
cur_original = resize(cur_original, (old_square_shape, old_square_shape))
|
||||
|
||||
reshape_cloak = cur_protected - cur_original
|
||||
start1, end1, start2, end2 = self.start_end_ls[i]
|
||||
|
||||
reshape_cloak = reshape_cloak[0:org_shape[0], 0:org_shape[1], :]
|
||||
reshape_cloak = cur_protected - cur_original
|
||||
reshape_cloak = reshape_cloak[start1:end1, start2:end2, :]
|
||||
|
||||
callback_id = self.callback_idx[i]
|
||||
bb = self.cropped_index[i]
|
||||
self.cloaked_faces[callback_id][bb[1]:bb[3], bb[0]:bb[2], :] += reshape_cloak
|
||||
self.cloaked_faces[callback_id][bb[0]:bb[2], bb[1]:bb[3], :] += reshape_cloak
|
||||
|
||||
for i in range(0, len(self.cloaked_faces)):
|
||||
self.cloaked_faces[i] = np.clip(self.cloaked_faces[i], 0.0, 255.0)
|
||||
return self.cloaked_faces
|
||||
return self.cloaked_faces, self.images_without_face
|
||||
|
||||
|
||||
def get_ends(longsize, window):
|
||||
start = (longsize - window) // 2
|
||||
end = start + window
|
||||
return start, end
|
||||
|
||||
|
||||
def dump_dictionary_as_json(dict, outfile):
|
||||
@ -251,17 +272,25 @@ def resize(img, sz):
|
||||
return im_data
|
||||
|
||||
|
||||
def init_gpu(gpu_index, force=False):
|
||||
if isinstance(gpu_index, list):
|
||||
gpu_num = ','.join([str(i) for i in gpu_index])
|
||||
def init_gpu(gpu):
|
||||
''' code to initialize gpu in tf2'''
|
||||
if isinstance(gpu, list):
|
||||
gpu_num = ','.join([str(i) for i in gpu])
|
||||
else:
|
||||
gpu_num = str(gpu_index)
|
||||
if "CUDA_VISIBLE_DEVICES" in os.environ and os.environ["CUDA_VISIBLE_DEVICES"] and not force:
|
||||
gpu_num = str(gpu)
|
||||
if "CUDA_VISIBLE_DEVICES" in os.environ:
|
||||
print('GPU already initiated')
|
||||
return
|
||||
os.environ["CUDA_VISIBLE_DEVICES"] = gpu_num
|
||||
sess = fix_gpu_memory()
|
||||
return sess
|
||||
gpus = tf.config.experimental.list_physical_devices('GPU')
|
||||
if gpus:
|
||||
try:
|
||||
tf.config.experimental.set_visible_devices(gpus[0], 'GPU')
|
||||
tf.config.experimental.set_memory_growth(gpus[0], True)
|
||||
logical_gpus = tf.config.experimental.list_logical_devices('GPU')
|
||||
print(len(gpus), "Physical GPUs,", len(logical_gpus), "Logical GPU")
|
||||
except RuntimeError as e:
|
||||
print(e)
|
||||
|
||||
|
||||
def fix_gpu_memory(mem_fraction=1):
|
||||
@ -398,28 +427,33 @@ def build_bottleneck_model(model, cut_off):
|
||||
|
||||
|
||||
def load_extractor(name):
|
||||
model_dir = os.path.join(os.path.expanduser('~'), '.fawkes')
|
||||
os.makedirs(model_dir, exist_ok=True)
|
||||
model_file = os.path.join(model_dir, "{}.h5".format(name))
|
||||
emb_file = os.path.join(model_dir, "{}_emb.p.gz".format(name))
|
||||
if os.path.exists(model_file):
|
||||
model = keras.models.load_model(model_file)
|
||||
else:
|
||||
print("Download models...")
|
||||
get_file("{}.h5".format(name), "http://mirror.cs.uchicago.edu/fawkes/files/{}.h5".format(name),
|
||||
cache_dir=model_dir, cache_subdir='')
|
||||
model = keras.models.load_model(model_file)
|
||||
hash_map = {"extractor_2": "ce703d481db2b83513bbdafa27434703",
|
||||
"extractor_0": "94854151fd9077997d69ceda107f9c6b"}
|
||||
assert name in ["extractor_2", 'extractor_0']
|
||||
model_file = pkg_resources.resource_filename("fawkes", "model/{}.h5".format(name))
|
||||
cur_hash = hash_map[name]
|
||||
model_dir = pkg_resources.resource_filename("fawkes", "model/")
|
||||
get_file("{}.h5".format(name), "http://mirror.cs.uchicago.edu/fawkes/files/{}.h5".format(name),
|
||||
cache_dir=model_dir, cache_subdir='', md5_hash=cur_hash)
|
||||
|
||||
if not os.path.exists(emb_file):
|
||||
get_file("{}_emb.p.gz".format(name), "http://mirror.cs.uchicago.edu/fawkes/files/{}_emb.p.gz".format(name),
|
||||
cache_dir=model_dir, cache_subdir='')
|
||||
|
||||
if hasattr(model.layers[-1], "activation") and model.layers[-1].activation == "softmax":
|
||||
raise Exception(
|
||||
"Given extractor's last layer is softmax, need to remove the top layers to make it into a feature extractor")
|
||||
model = keras.models.load_model(model_file)
|
||||
model = Extractor(model)
|
||||
return model
|
||||
|
||||
|
||||
class Extractor(object):
|
||||
def __init__(self, model):
|
||||
self.model = model
|
||||
|
||||
def predict(self, imgs):
|
||||
imgs = imgs / 255.0
|
||||
embeds = l2_norm(self.model(imgs))
|
||||
return embeds
|
||||
|
||||
def __call__(self, x):
|
||||
return self.predict(x)
|
||||
|
||||
|
||||
def get_dataset_path(dataset):
|
||||
model_dir = os.path.join(os.path.expanduser('~'), '.fawkes')
|
||||
if not os.path.exists(os.path.join(model_dir, "config.json")):
|
||||
@ -496,6 +530,7 @@ def select_target_label(imgs, feature_extractors_ls, feature_extractors_names, m
|
||||
max_id = random.choice(max_id_ls[:20])
|
||||
|
||||
target_data_id = paths[int(max_id)]
|
||||
target_data_id = 9
|
||||
print("target ID: {}".format(target_data_id))
|
||||
|
||||
image_dir = os.path.join(model_dir, "target_data/{}".format(target_data_id))
|
||||
@ -517,8 +552,8 @@ def select_target_label(imgs, feature_extractors_ls, feature_extractors_names, m
|
||||
target_images = [image.img_to_array(image.load_img(cur_path)) for cur_path in
|
||||
image_paths]
|
||||
|
||||
target_images = np.array([resize(x, (224, 224)) for x in target_images])
|
||||
target_images = preprocess(target_images, 'imagenet')
|
||||
target_images = np.array([resize(x, (IMG_SIZE, IMG_SIZE)) for x in target_images])
|
||||
target_images = preprocess(target_images, PREPROCESS)
|
||||
|
||||
target_images = list(target_images)
|
||||
while len(target_images) < len(imgs):
|
||||
@ -528,6 +563,13 @@ def select_target_label(imgs, feature_extractors_ls, feature_extractors_names, m
|
||||
return np.array(target_images)
|
||||
|
||||
|
||||
def l2_norm(x, axis=1):
|
||||
"""l2 norm"""
|
||||
norm = tf.norm(x, axis=axis, keepdims=True)
|
||||
output = x / norm
|
||||
return output
|
||||
|
||||
|
||||
""" TensorFlow implementation get_file
|
||||
https://github.com/tensorflow/tensorflow/blob/v2.3.0/tensorflow/python/keras/utils/data_utils.py#L168-L297
|
||||
"""
|
||||
@ -544,16 +586,18 @@ def get_file(fname,
|
||||
archive_format='auto',
|
||||
cache_dir=None):
|
||||
if cache_dir is None:
|
||||
cache_dir = os.path.join(os.path.expanduser('~'), '.fawkes')
|
||||
cache_dir = os.path.join(os.path.expanduser('~'), '.keras')
|
||||
if md5_hash is not None and file_hash is None:
|
||||
file_hash = md5_hash
|
||||
hash_algorithm = 'md5'
|
||||
datadir_base = os.path.expanduser(cache_dir)
|
||||
if not os.access(datadir_base, os.W_OK):
|
||||
datadir_base = os.path.join('/tmp', '.fawkes')
|
||||
datadir_base = os.path.join('/tmp', '.keras')
|
||||
datadir = os.path.join(datadir_base, cache_subdir)
|
||||
_makedirs_exist_ok(datadir)
|
||||
|
||||
# fname = path_to_string(fname)
|
||||
|
||||
if untar:
|
||||
untar_fpath = os.path.join(datadir, fname)
|
||||
fpath = untar_fpath + '.tar.gz'
|
||||
@ -561,12 +605,35 @@ def get_file(fname,
|
||||
fpath = os.path.join(datadir, fname)
|
||||
|
||||
download = False
|
||||
if not os.path.exists(fpath):
|
||||
if os.path.exists(fpath):
|
||||
# File found; verify integrity if a hash was provided.
|
||||
if file_hash is not None:
|
||||
if not validate_file(fpath, file_hash, algorithm=hash_algorithm):
|
||||
print('A local file was found, but it seems to be '
|
||||
'incomplete or outdated because the ' + hash_algorithm +
|
||||
' file hash does not match the original value of ' + file_hash +
|
||||
' so we will re-download the data.')
|
||||
download = True
|
||||
else:
|
||||
download = True
|
||||
|
||||
if download:
|
||||
print('Downloading data from', origin)
|
||||
|
||||
class ProgressTracker(object):
|
||||
# Maintain progbar for the lifetime of download.
|
||||
# This design was chosen for Python 2.7 compatibility.
|
||||
progbar = None
|
||||
|
||||
def dl_progress(count, block_size, total_size):
|
||||
if ProgressTracker.progbar is None:
|
||||
if total_size == -1:
|
||||
total_size = None
|
||||
ProgressTracker.progbar = Progbar(total_size)
|
||||
else:
|
||||
ProgressTracker.progbar.update(count * block_size)
|
||||
|
||||
error_msg = 'URL fetch failure on {}: {} -- {}'
|
||||
dl_progress = None
|
||||
try:
|
||||
try:
|
||||
urlretrieve(origin, fpath, dl_progress)
|
||||
@ -578,7 +645,7 @@ def get_file(fname,
|
||||
if os.path.exists(fpath):
|
||||
os.remove(fpath)
|
||||
raise
|
||||
# ProgressTracker.progbar = None
|
||||
ProgressTracker.progbar = None
|
||||
|
||||
if untar:
|
||||
if not os.path.exists(untar_fpath):
|
||||
@ -632,3 +699,53 @@ def _makedirs_exist_ok(datadir):
|
||||
raise
|
||||
else:
|
||||
os.makedirs(datadir, exist_ok=True) # pylint: disable=unexpected-keyword-arg
|
||||
|
||||
|
||||
def validate_file(fpath, file_hash, algorithm='auto', chunk_size=65535):
|
||||
"""Validates a file against a sha256 or md5 hash.
|
||||
Arguments:
|
||||
fpath: path to the file being validated
|
||||
file_hash: The expected hash string of the file.
|
||||
The sha256 and md5 hash algorithms are both supported.
|
||||
algorithm: Hash algorithm, one of 'auto', 'sha256', or 'md5'.
|
||||
The default 'auto' detects the hash algorithm in use.
|
||||
chunk_size: Bytes to read at a time, important for large files.
|
||||
Returns:
|
||||
Whether the file is valid
|
||||
"""
|
||||
if (algorithm == 'sha256') or (algorithm == 'auto' and len(file_hash) == 64):
|
||||
hasher = 'sha256'
|
||||
else:
|
||||
hasher = 'md5'
|
||||
|
||||
if str(_hash_file(fpath, hasher, chunk_size)) == str(file_hash):
|
||||
return True
|
||||
else:
|
||||
return False
|
||||
|
||||
|
||||
def _hash_file(fpath, algorithm='sha256', chunk_size=65535):
|
||||
"""Calculates a file sha256 or md5 hash.
|
||||
Example:
|
||||
```python
|
||||
_hash_file('/path/to/file.zip')
|
||||
'e3b0c44298fc1c149afbf4c8996fb92427ae41e4649b934ca495991b7852b855'
|
||||
```
|
||||
Arguments:
|
||||
fpath: path to the file being validated
|
||||
algorithm: hash algorithm, one of `'auto'`, `'sha256'`, or `'md5'`.
|
||||
The default `'auto'` detects the hash algorithm in use.
|
||||
chunk_size: Bytes to read at a time, important for large files.
|
||||
Returns:
|
||||
The file hash
|
||||
"""
|
||||
if (algorithm == 'sha256') or (algorithm == 'auto' and len(hash) == 64):
|
||||
hasher = hashlib.sha256()
|
||||
else:
|
||||
hasher = hashlib.md5()
|
||||
|
||||
with open(fpath, 'rb') as fpath_file:
|
||||
for chunk in iter(lambda: fpath_file.read(chunk_size), b''):
|
||||
hasher.update(chunk)
|
||||
|
||||
return hasher.hexdigest()
|
||||
|
66
master.py
66
master.py
@ -1,66 +0,0 @@
|
||||
import socket
|
||||
import subprocess
|
||||
import sys
|
||||
import time
|
||||
|
||||
print(socket.gethostname())
|
||||
|
||||
|
||||
def assign_gpu(args, gpu_idx):
|
||||
for i, arg in enumerate(args):
|
||||
if arg == "GPUID":
|
||||
args[i] = str(gpu_idx)
|
||||
return args
|
||||
|
||||
|
||||
def produce_present():
|
||||
process_ls = []
|
||||
gpu_ls = list(sys.argv[1])
|
||||
max_num = int(sys.argv[2])
|
||||
|
||||
available_gpus = []
|
||||
i = 0
|
||||
while len(available_gpus) < max_num:
|
||||
if i > len(gpu_ls) - 1:
|
||||
i = 0
|
||||
available_gpus.append(gpu_ls[i])
|
||||
i += 1
|
||||
|
||||
process_dict = {}
|
||||
all_queries_to_run = []
|
||||
|
||||
for m in ['mid', 'low', 'min']:
|
||||
for directory in ['KimKardashian', 'Liuyifei', 'Obama', 'TaylorSwift', 'TomHolland']:
|
||||
args = ['python3', 'protection.py', '--gpu', 'GPUID', '-d',
|
||||
'/home/shansixioing/fawkes/data/test/{}/'.format(directory),
|
||||
'--batch-size', '30', '-m', m,
|
||||
'--debug']
|
||||
args = [str(x) for x in args]
|
||||
all_queries_to_run.append(args)
|
||||
|
||||
for args in all_queries_to_run:
|
||||
cur_gpu = available_gpus.pop(0)
|
||||
args = assign_gpu(args, cur_gpu)
|
||||
print(" ".join(args))
|
||||
p = subprocess.Popen(args)
|
||||
process_ls.append(p)
|
||||
process_dict[p] = cur_gpu
|
||||
|
||||
gpu_ls.append(cur_gpu)
|
||||
time.sleep(5)
|
||||
while not available_gpus:
|
||||
for p in process_ls:
|
||||
poll = p.poll()
|
||||
if poll is not None:
|
||||
process_ls.remove(p)
|
||||
available_gpus.append(process_dict[p])
|
||||
|
||||
time.sleep(20)
|
||||
|
||||
|
||||
def main():
|
||||
produce_present()
|
||||
|
||||
|
||||
if __name__ == '__main__':
|
||||
main()
|
14
setup.py
14
setup.py
@ -75,10 +75,10 @@ class DeployCommand(Command):
|
||||
setup_requires = []
|
||||
|
||||
install_requires = [
|
||||
'numpy==1.16.4',
|
||||
# 'tensorflow-gpu>=1.13.1, <=1.14.0',
|
||||
'tensorflow>=1.12.0, <=1.15.0', # change this is tensorflow-gpu if using GPU machine.
|
||||
'keras>=2.2.5, <=2.3.1',
|
||||
'numpy>=1.19.5',
|
||||
'tensorflow>=2.0.0',
|
||||
'keras>=2.3.1',
|
||||
'mtcnn',
|
||||
'pillow>=7.0.0',
|
||||
'bleach>=2.1.0'
|
||||
]
|
||||
@ -92,8 +92,8 @@ setup(
|
||||
long_description_content_type='text/markdown',
|
||||
url="https://github.com/Shawn-Shan/fawkes",
|
||||
author='Shawn Shan',
|
||||
author_email='shansixiong@cs.uchicago.edu',
|
||||
keywords='fawkes privacy clearview',
|
||||
author_email='shawnshan@cs.uchicago.edu',
|
||||
keywords='fawkes privacy ML',
|
||||
classifiers=[
|
||||
'Development Status :: 3 - Alpha',
|
||||
'License :: OSI Approved :: MIT License',
|
||||
@ -112,5 +112,5 @@ setup(
|
||||
},
|
||||
include_package_data=True,
|
||||
zip_safe=False,
|
||||
python_requires='>=3.5,<3.8',
|
||||
python_requires='>=3.5',
|
||||
)
|
||||
|
Loading…
Reference in New Issue
Block a user